Python for Computational Science
Last updated: May 2010
Contents
Intro to Python programming
Array computing and visualizationMore about array computingIntro to mixed language programmingIntro to GUI programmingNumerical mixed-language programmingQuick Python review

Intro to Python programming

Make sure you have the software
 | Python version 2.5
|
 | Numerical Python (numpy)
|
 | Gnuplot program, Python Gnuplot module
|
 | SciTools
|
 | For multi-language programming: gcc, g++, g77
|
 | For GUI programming: Tcl/Tk, Pmw
|
 | Some Python modules are handy: IPython, Epydoc, ...
|

Material associated with these slides
 | These slides have a companion book:
Scripting in Computational Science, 3rd edition,
Texts in Computational Science and Engineering,
Springer, 2008
|
 | All examples can be downloaded as a tarfile
http://folk.uio.no/hpl/scripting/TCSE3-3rd-examples.tar.gz
|
 | Software associated with the book and slides: SciTools
http://code.google.com/p/scitools/
|

Installing TCSE3-3rd-examples.tar.gz
 |
Pack TCSE3-3rd-examples.tar.gz out in a directory and let scripting be an environment variable pointing to the top directory:
tar xvzf TCSE3-3rd-examples.tar.gz
export scripting=`pwd`
All paths in these slides are given relative to scripting, e.g., src/py/intro/hw.py is reached as
$scripting/src/py/intro/hw.py
|

Scientific Hello World script
 | All computer languages intros start with a program that
prints "Hello, World!" to the screen
|
 | Scientific computing extension: read a number, compute its sine
value, and print out
|
 | The script, called hw.py, should be run like this:
python hw.py 3.4
or just (Unix)
./hw.py 3.4
|
 | Output:
Hello, World! sin(3.4)=-0.255541102027
|

Purpose of this script
Demonstrate
 | how to get input from the command line
|
 | how to call a math function like sin(x)
|
 | how to work with variables
|
 | how to print text and numbers
|

The code
 | File hw.py:
#!/usr/bin/env python
# load system and math module:
import sys, math
# extract the 1st command-line argument:
r = float(sys.argv[1])
s = math.sin(r)
print "Hello, World! sin(" + str(r) + ")=" + str(s)
|
 | Make the file executable (on Unix):
chmod a+rx hw.py
|

Comments
 | The first line specifies the interpreter of the script
(here the first python program in your path)
python hw.py 1.4 # first line is treated as comment
./hw.py 1.4 # first line is used to specify an interpreter
|
 | Even simple scripts must load modules:
import sys, math
|
 | Numbers and strings are two different types:
r = sys.argv[1] # r is string
s = math.sin(float(r))
# sin expects number, not string r
# s becomes a floating-point number
|

Alternative print statements
 | Desired output:
Hello, World! sin(3.4)=-0.255541102027
|
 | String concatenation:
print "Hello, World! sin(" + str(r) + ")=" + str(s)
|
 | printf-like statement:
print "Hello, World! sin(%g)=%g" % (r,s)
|
 | Variable interpolation:
print "Hello, World! sin(%(r)g)=%(s)g" % vars()
|

printf format strings
%d : integer
%5d : integer in a field of width 5 chars
%-5d : integer in a field of width 5 chars,
but adjusted to the left
%05d : integer in a field of width 5 chars,
padded with zeroes from the left
%g : float variable in %f or %g notation
%e : float variable in scientific notation
%11.3e : float variable in scientific notation,
with 3 decimals, field of width 11 chars
%5.1f : float variable in fixed decimal notation,
with one decimal, field of width 5 chars
%.3f : float variable in fixed decimal form,
with three decimals, field of min. width
%s : string
%-20s : string in a field of width 20 chars,
and adjusted to the left

Strings in Python
 | Single- and double-quoted strings work in the same way
s1 = "some string with a number %g" % r
s2 = 'some string with a number %g' % r # = s1
|
 | Triple-quoted strings can be multi line with embedded
newlines:
text = """
large portions of a text
can be conveniently placed
inside triple-quoted strings
(newlines are preserved)"""
|
 | Raw strings, where backslash is backslash:
s3 = r'\(\s+\.\d+\)'
# with ordinary string (must quote backslash):
s3 = '\\(\\s+\\.\\d+\\)'
|

Where to find Python info
 | Make a bookmark for \$scripting/doc.html
|
 | Follow link to Index to Python Library Reference
(complete on-line Python reference)
|
 | Click on Python keywords, modules etc.
|
 | Online alternative: pydoc, e.g., pydoc math
|
 | pydoc lists all classes and functions in a module
|
 | Alternative: Python in a Nutshell (or Beazley's textbook)
|
 | Recommendation: use these slides and associated book together with
the Python Library Reference, and learn by doing exercises
|

New example: reading/writing data files
Tasks:
 | Read (x,y) data from a two-column file
|
 | Transform y values to f(y)
|
 | Write (x,f(y)) to a new file
|
What to learn:
 | How to open, read, write and close files
|
 | How to write and call a function
|
 | How to work with arrays (lists)
|
File: src/py/intro/datatrans1.py

Reading input/output filenames
 | Usage:
./datatrans1.py infilename outfilename
|
 | Read the two command-line arguments:
input and output filenames
infilename = sys.argv[1]
outfilename = sys.argv[2]
|
 | Command-line arguments are in sys.argv[1:]
|
 | sys.argv[0] is the name of the script
|

Exception handling
 | What if the user fails to provide two command-line arguments?
|
 | Python aborts execution with an informative error message
|
 | A good alternative is to handle the error manually inside the program code:
try:
infilename = sys.argv[1]
outfilename = sys.argv[2]
except:
# try block failed,
# we miss two command-line arguments
print 'Usage:', sys.argv[0], 'infile outfile'
sys.exit(1)
This is the common way of dealing with errors in Python,
called exception handling
|

Open file and read line by line
 | Open files:
ifile = open( infilename, 'r') # r for reading
ofile = open(outfilename, 'w') # w for writing
afile = open(appfilename, 'a') # a for appending
|
 | Read line by line:
for line in ifile:
# process line
|
 | Observe: blocks are indented; no braces!
|

Defining a function
import math
def myfunc(y):
if y >= 0.0:
return y**5*math.exp(-y)
else:
return 0.0
# alternative way of calling module functions
# (gives more math-like syntax in this example):
from math import *
def myfunc(y):
if y >= 0.0:
return y**5*exp(-y)
else:
return 0.0

Data transformation loop
 | Input file format: two columns with numbers
0.1 1.4397
0.2 4.325
0.5 9.0
|
 | Read a line with x and y, transform y, write x and f(y):
for line in ifile:
pair = line.split()
x = float(pair[0]); y = float(pair[1])
fy = myfunc(y) # transform y value
ofile.write('%g %12.5e\n' % (x,fy))
|

Alternative file reading
 | This construction is more flexible and traditional in Python (and a bit strange...):
while 1:
line = ifile.readline() # read a line
if not line: break # end of file: jump out of loop
# process line
i.e., an 'infinite' loop with the termination criterion
inside the loop
|

Loading data into lists
 | Read input file into list of lines:
lines = ifile.readlines()
|
 | Now the 1st line is lines[0], the 2nd is lines[1], etc.
|
 | Store x and y data in lists:
# go through each line,
# split line into x and y columns
x = []; y = [] # store data pairs in lists x and y
for line in lines:
xval, yval = line.split()
x.append(float(xval))
y.append(float(yval))
|
See src/py/intro/datatrans2.py for this version

Loop over list entries
 | For-loop in Python:
for i in range(start,stop,inc):
...
for j in range(stop):
...
generates
i = start, start+inc, start+2*inc, ..., stop-1
j = 0, 1, 2, ..., stop-1
|
 | Loop over (x,y) values:
ofile = open(outfilename, 'w') # open for writing
for i in range(len(x)):
fy = myfunc(y[i]) # transform y value
ofile.write('%g %12.5e\n' % (x[i], fy))
ofile.close()
|

Running the script
 | Method 1: write just the name of the scriptfile:
./datatrans1.py infile outfile
# or
datatrans1.py infile outfile
if . (current working directory) or the directory containing
datatrans1.py is in the path
|
 | Method 2: run an interpreter explicitly:
python datatrans1.py infile outfile
Use the first python program found in the path |
 | This works on Windows too (method 1 requires the right assoc/ftype bindings for .py files)
|

More about headers
 | In method 1, the interpreter to be used is specified in the first line
|
 | Explicit path to the interpreter:
#!/usr/local/bin/python
or perhaps your own Python interpreter:
#!/home/hpl/projects/scripting/Linux/bin/python
|
 | Using env to find the first Python interpreter in the path:
#!/usr/bin/env python
|

Are scripts compiled?
 | Yes and no, depending on how you see it
|
 | Python first compiles the script into bytecode
|
 | The bytecode is then interpreted
|
 | No linking with libraries; libraries are imported dynamically when needed
|
 | It appears as there is no compilation
|
 | Quick development: just edit the script and run!
(no time-consuming compilation and linking)
|
 | Extensive error checking at run time
|

About Python for the experienced computer scientist
 | Everything in Python is an object (number, function, list, file,
module, class, socket, ...)
|
 | Objects are instances of a class -- lots of classes are defined (float, int, list, file, ...) and the programmer can define new classes
|
 | Variables are names for (or ``pointers'' or ``references'' to) objects:
A = 1 # make an int object with value 1 and name A
A = 'Hi!' # make a str object with value 'Hi!' and name A
print A[1] # A[1] is a str object 'i', print this object
A = [-1,1] # let A refer to a list object with 2 elements
A[-1] = 2 # change the list A refers to in-place
b = A # let name b refer to the same object as A
print b # results in the string '[-1, 2]'
|
 | Functions are either stand-alone or part of classes:
n = len(A) # len(somelist) is a stand-alone function
A.append(4) # append is a list method (function)
|

Python and error checking
 | Easy to introduce intricate bugs?
 | no declaration of variables
|  | functions can "eat anything"
|
|
 | No, extensive consistency checks at run time replace the need for strong typing and compile-time checks
|
 | Example: sending a string to the sine function, math.sin('t'), triggers a run-time error (type incompatibility)
|
 | Example: try to open a non-existing file
./datatrans1.py qqq someoutfile
Traceback (most recent call last):
File "./datatrans1.py", line 12, in ?
ifile = open( infilename, 'r')
IOError:[Errno 2] No such file or directory:'qqq'
|

Computing with arrays
 | x and y in datatrans2.py are lists
|
 | We can compute with lists element by element (as shown)
|
 | However: using Numerical Python (NumPy) arrays instead of lists is
much more efficient and convenient
|
 | Numerical Python is an extension of Python: a new fixed-size
array type and lots of functions operating on such arrays
|

A first glimpse of NumPy
 | Import (more on this later...):
from numpy import *
x = linspace(0, 1, 1001) # 1001 values between 0 and 1
x = sin(x) # computes sin(x[0]), sin(x[1]) etc.
|
 | x=sin(x) is 13 times faster than an explicit loop:
for i in range(len(x)):
x[i] = sin(x[i])
because sin(x) invokes an efficient loop in C
|

Loading file data into NumPy arrays
 | A special module loads tabular file data into NumPy arrays:
import scitools.filetable
f = open(infilename, 'r')
x, y = scitools.filetable.read_columns(f)
f.close()
|
 | Now we can compute with the NumPy arrays x and y:
x = 10*x
y = 2*y + 0.1*sin(x)
|
 | We can easily write x and y back to a file:
f = open(outfilename, 'w')
scitools.filetable.write_columns(f, x, y)
f.close()
|

More on computing with NumPy arrays
 | Multi-dimensional arrays can be constructed:
x = zeros(n) # array with indices 0,1,...,n-1
x = zeros((m,n)) # two-dimensional array
x[i,j] = 1.0 # indexing
x = zeros((p,q,r)) # three-dimensional array
x[i,j,k] = -2.1
x = sin(x)*cos(x)
|
 | We can plot one-dimensional arrays:
from scitools.easyviz import * # plotting
x = linspace(0, 2, 21)
y = x + sin(10*x)
plot(x, y)
|
 | NumPy has lots of math functions and operations
|
 | SciPy is a comprehensive extension of NumPy
|
 | NumPy + SciPy is a kind of Matlab replacement for many people
|

Interactive Python
 | Python statements can be run interactively in a Python shell
|
 | The ``best'' shell is called IPython
|
 | Sample session with IPython:
Unix/DOS> ipython
...
In [1]:3*4-1
Out[1]:11
In [2]:from math import *
In [3]:x = 1.2
In [4]:y = sin(x)
In [5]:x
Out[5]:1.2
In [6]:y
Out[6]:0.93203908596722629
|

Editing capabilities in IPython
 | Up- and down-arrays: go through command history
|
 | Emacs key bindings for editing previous commands
|
 | The underscore variable holds the last output
In [6]:y
Out[6]:0.93203908596722629
In [7]:_ + 1
Out[7]:1.93203908596722629
|

TAB completion
 | IPython supports TAB completion: write a part of a command or
name (variable, function, module), hit the TAB key, and IPython will
complete the word or show different alternatives:
In [1]: import math
In [2]: math.<TABKEY>
math.__class__ math.__str__ math.frexp
math.__delattr__ math.acos math.hypot
math.__dict__ math.asin math.ldexp
...
or
In [2]: my_variable_with_a_very_long_name = True
In [3]: my<TABKEY>
In [3]: my_variable_with_a_very_long_name
You can increase your typing speed with TAB completion!
|

More examples
In [1]:f = open('datafile', 'r')
IOError: [Errno 2] No such file or directory: 'datafile'
In [2]:f = open('.datatrans_infile', 'r')
In [3]:from scitools.filetable import read_columns
In [4]:x, y = read_columns(f)
In [5]:x
Out[5]:array([ 0.1, 0.2, 0.3, 0.4])
In [6]:y
Out[6]:array([ 1.1 , 1.8 , 2.22222, 1.8 ])

IPython and the Python debugger
 | Scripts can be run from IPython:
In [1]:run scriptfile arg1 arg2 ...
e.g.,
In [1]:run datatrans2.py .datatrans_infile tmp1
|
 | IPython is integrated with Python's pdb debugger
|
 | pdb can be automatically invoked when an exception occurs:
In [29]:%pdb on # invoke pdb automatically
In [30]:run datatrans2.py infile tmp2
|

More on debugging
 | This happens when the infile name is wrong:
/home/work/scripting/src/py/intro/datatrans2.py
7 print "Usage:",sys.argv[0], "infile outfile"; sys.exit(1)
8
----> 9 ifile = open(infilename, 'r') # open file for reading
10 lines = ifile.readlines() # read file into list of lines
11 ifile.close()
IOError: [Errno 2] No such file or directory: 'infile'
> /home/work/scripting/src/py/intro/datatrans2.py(9)?()
-> ifile = open(infilename, 'r') # open file for reading
(Pdb) print infilename
infile
|

On the efficiency of scripts
Consider datatrans1.py: read 100 000 (x,y) data from a pure text (ASCII)
file and write (x,f(y)) out again
 | Pure Python: 4s
|
 | Pure Perl: 3s
|
 | Pure Tcl: 11s
|
 | Pure C (fscanf/fprintf): 1s
|
 | Pure C++ (iostream): 3.6s
|
 | Pure C++ (buffered streams): 2.5s
|
 | Numerical Python modules: 2.2s (!)
|
(Computer: IBM X30, 1.2 GHz, 512 Mb RAM, Linux,
gcc 3.3)

The classical script
 | Simple, classical Unix shell scripts are widely used to replace sequences of manual steps in a terminal window
|
 | Such scripts are crucial for scientific reliability and human efficiency!
|
 | Shell script newbie? Wake up and adapt this example to your projects!
|
 | Typical situation in computer simulation:
 | run a simulation program with some input
|  | run a visualization program and produce graphs
|
|
 | Programs are supposed to run from the command line, with input from files or from command-line arguments
|
 | We want to automate the manual steps by a Python script
|

What to learn
 | Parsing command-line options:
somescript -option1 value1 -option2 value2
|
 | Removing and creating directories
|
 | Writing data to file
|
 | Running stand-alone programs (applications)
|

A code: simulation of an oscillating system
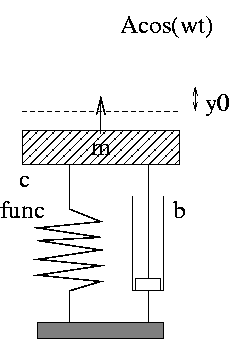
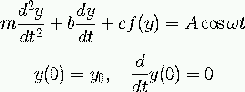
Code: oscillator (written in Fortran 77)

Usage of the simulation code
 |
Input: m, b, c, and so on read from standard input
|
 | How to run the code:
oscillator < file
where file can be
3.0
0.04
1.0
...
i.e., values of m, b, c, etc. -- in the right order!
|
 | The resulting time series y(t) is stored in a file
sim.dat with t and y(t) in the 1st and 2nd column, respectively
|

A plot of the solution
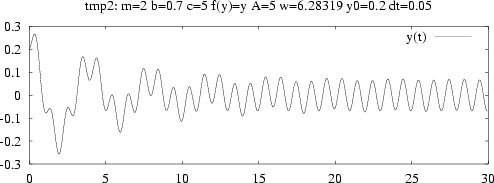

Plotting graphs in Gnuplot
 | Commands:
set title 'case: m=3 b=0.7 c=1 f(y)=y A=5 ...';
# screen plot: (x,y) data are in the file sim.dat
plot 'sim.dat' title 'y(t)' with lines;
# hardcopies:
set size ratio 0.3 1.5, 1.0;
set term postscript eps mono dashed 'Times-Roman' 28;
set output 'case.ps';
plot 'sim.dat' title 'y(t)' with lines;
# make a plot in PNG format as well:
set term png small;
set output 'case.png';
plot 'sim.dat' title 'y(t)' with lines;
|
 | Commands can be given interactively or put in a file
|

Typical manual work
 | Change physical or numerical parameters by editing the
simulator's input file
|
 | Run simulator:
oscillator < inputfile
|
 | Edit plot commands in the file case.gp
|
 | Make plot:
gnuplot -persist -geometry 800x200 case.gp
|
 | Plot annotations in case.gp must be consistent with inputfile
|
 | Let's automate!
|
 | You can easily adapt this example to your own work!
|
Final script: src/py/intro/simviz1.py

The user interface
 | Usage:
./simviz1.py -m 3.2 -b 0.9 -dt 0.01 -case run1
Sensible default values for all options
|
 | Put simulation and plot files in a subdirectory
(specified by -case run1)
|

Program tasks
 | Set default values of m, b, c etc.
|
 | Parse command-line options (-m, -b etc.) and assign new values
to m, b, c etc.
|
 | Create and move to subdirectory
|
 | Write input file for the simulator
|
 | Run simulator
|
 | Write Gnuplot commands in a file
|
 | Run Gnuplot
|

Parsing command-line options
 | Set default values of the script's input parameters:
m = 1.0; b = 0.7; c = 5.0; func = 'y'; A = 5.0;
w = 2*math.pi; y0 = 0.2; tstop = 30.0; dt = 0.05;
case = 'tmp1'; screenplot = 1
|
 | Examine command-line options in sys.argv:
# read variables from the command line, one by one:
while len(sys.argv) >= 2:
option = sys.argv[1]; del sys.argv[1]
if option == '-m':
m = float(sys.argv[1]); del sys.argv[1]
elif option == '-b':
b = float(sys.argv[1]); del sys.argv[1]
...
Note: sys.argv[1] is text, but we may want a float for numerical operations
|

Modules for parsing command-line arguments
 | Python offers two modules for command-line argument parsing: getopt
and optparse
|
 | These accept short options (-m) and long options (--mass)
|
 | getopt examines the command line and returns pairs of options and values ((--mass, 2.3))
|
 | optparse is a bit more comprehensive to use and makes the command-line options available as attributes in an object
|
 | In this introductory example we rely on manual parsing since this exemplifies basic Python programming
|

Creating a subdirectory
 | Python has a rich cross-platform operating system (OS) interface
|
 | Skip Unix- or DOS-specific commands; do all OS operations in Python!
|
 | Safe creation of a subdirectory:
dir = case # subdirectory name
import os, shutil
if os.path.isdir(dir): # does dir exist?
shutil.rmtree(dir) # yes, remove old files
os.mkdir(dir) # make dir directory
os.chdir(dir) # move to dir
|

Writing the input file to the simulator
f = open('%s.i' % case, 'w')
f.write("""
%(m)g
%(b)g
%(c)g
%(func)s
%(A)g
%(w)g
%(y0)g
%(tstop)g
%(dt)g
""" % vars())
f.close()
Note: triple-quoted string for multi-line output

Running the simulation
 | Stand-alone programs can be run as
failure = os.system(command)
# or
import commands
failure, output = commands.getstatusoutput(command)
|
 | output contains the output of command that in case of os.system will be printed in the terminal window
|
 | failure is 0 (false) for a successful run of command
|
 | Our use:
cmd = 'oscillator < %s.i' % case # command to run
import commands
failure, output = commands.getstatusoutput(cmd)
if failure:
print 'running the oscillator code failed'
print output
sys.exit(1)
|

Making plots
 | Make Gnuplot script:
f = open(case + '.gnuplot', 'w')
f.write("""
set title '%s: m=%g b=%g c=%g f(y)=%s A=%g ...';
...
...
""" % (case,m,b,c,func,A,w,y0,dt,case,case))
...
f.close()
|
 | Run Gnuplot:
cmd = 'gnuplot -geometry 800x200 -persist ' \
+ case + '.gnuplot'
failure, output = commands.getstatusoutput(cmd)
if failure:
print 'running gnuplot failed'; print output; sys.exit(1)
|

Python vs Unix shell script
 | Our simviz1.py script is traditionally written as a Unix shell script
|
 | What are the advantages of using Python here?
 | Easier command-line parsing
|  | Runs on Windows and Mac as well as Unix
|  | Easier extensions (loops, storing data in arrays, analyzing results, etc.)
|
|
Example on corresponding Bash script file: src/bash/simviz1.sh

Other programs for curve plotting
 | It is easy to replace Gnuplot by another plotting program
|
 | Matlab, for instance:
f = open(case + '.m', 'w') # write to Matlab M-file
# (the character % must be written as %% in printf-like strings)
f.write("""
load sim.dat %% read sim.dat into sim matrix
plot(sim(:,1),sim(:,2)) %% plot 1st column as x, 2nd as y
legend('y(t)')
title('%s: m=%g b=%g c=%g f(y)=%s A=%g w=%g y0=%g dt=%g')
outfile = '%s.ps'; print('-dps', outfile) %% ps BW plot
outfile = '%s.png'; print('-dpng', outfile) %% png color plot
""" % (case,m,b,c,func,A,w,y0,dt,case,case))
if screenplot: f.write('pause(30)\n')
f.write('exit\n'); f.close()
if screenplot:
cmd = 'matlab -nodesktop -r ' + case + ' > /dev/null &'
else:
cmd = 'matlab -nodisplay -nojvm -r ' + case
failure, output = commands.getstatusoutput(cmd)
|

Series of numerical experiments
 | Suppose we want to run a series of experiments with different m values
|
 | Put a script on top of simviz1.py,
./loop4simviz1.py m_min m_max dm \
[options as for simviz1.py]
with a loop over m, which calls simviz1.py inside the loop
|
 | Each experiment is archived in a separate directory
|
 | That is, loop4simviz1.py controls the -m and -case options to simviz1.py
|

Handling command-line args (1)
 | The first three arguments define the m values:
try:
m_min = float(sys.argv[1])
m_max = float(sys.argv[2])
dm = float(sys.argv[3])
except:
print 'Usage:',sys.argv[0],\
'm_min m_max m_increment [ simviz1.py options ]'
sys.exit(1)
|
 | Pass the rest of the arguments, sys.argv[4:], to simviz1.py
|
 | Problem: sys.argv[4:] is a list, we need a string
['-b','5','-c','1.1'] -> '-b 5 -c 1.1'
|

Handling command-line args (2)
 | ' '.join(list) can make a string out of the list list, with a blank between
each item
simviz1_options = ' '.join(sys.argv[4:])
|
 | Example:
./loop4simviz1.py 0.5 2 0.5 -b 2.1 -A 3.6
results in the same as
m_min = 0.5
m_max = 2.0
dm = 0.5
simviz1_options = '-b 2.1 -A 3.6'
|

The loop over m
 | Cannot use
for m in range(m_min, m_max, dm):
because range works with integers only
|
 | A while-loop is appropriate:
m = m_min
while m <= m_max:
case = 'tmp_m_%g' % m
s = 'python simviz1.py %s -m %g -case %s' % \
(simviz1_options, m, case)
failure, output = commands.getstatusoutput(s)
m += dm
(Note: our -m and -case will override any -m or
-case option provided by the user)
|

Collecting plots in an HTML file
 | Many runs of simviz1.py can be automated, many results are generated, and we need a way to browse the results
|
 | Idea: collect all plots in a common HTML file and let the script automate the writing of the HTML file
html = open('tmp_mruns.html', 'w')
html.write('<HTML><BODY BGCOLOR="white">\n')
m = m_min
while m <= m_max:
case = 'tmp_m_%g' % m
cmd = 'python simviz1.py %s -m %g -case %s' % \
(simviz1_options, m, case)
failure, output = commands.getstatusoutput(cmd)
html.write('<H1>m=%g</H1> <IMG SRC="%s">\n' \
% (m,os.path.join(case,case+'.png')))
m += dm
html.write('</BODY></HTML>\n')
|
 | Only 4 additional statements!
|

Animated GIF file
 | When we vary m, wouldn't it be nice to see progressive plots put together in a movie?
|
 | Can combine the PNG files together in an animated GIF file:
convert -delay 50 -loop 1000 -crop 0x0 \
plot1.png plot2.png plot3.png plot4.png ... movie.gif
animate movie.gif # or display movie.gif
(convert and animate are ImageMagick tools)
|
 | Collect all PNG filenames in a list and join the list items to form the convert arguments
|
 | Run the convert program
|

Some improvements
 | Enable loops over an arbitrary parameter (not only m)
'-m %g' % m
# is replaced with
'-%s %s' % (str(prm_name), str(prm_value))
# prm_value plays the role of the m variable
# prm_name ('m', 'b', 'c', ...) is read as input
|
 | New feature: keep the range of the y axis fixed (for movie)
|
 | Files:
simviz1.py : run simulation and visualization
simviz2.py : additional option for yaxis scale
loop4simviz1.py : m loop calling simviz1.py
loop4simviz2.py : loop over any parameter in
simviz2.py and make movie
|

Playing around with experiments
We can perform lots of different experiments:
 | Study the impact of increasing the mass:
./loop4simviz2.py m 0.1 6.1 0.5 -yaxis -0.5 0.5 -noscreenplot
|
 | Study the impact of a nonlinear spring:
./loop4simviz2.py c 5 30 2 -yaxis -0.7 0.7 -b 0.5 \
-func siny -noscreenplot
|
 | Study the impact of increasing the damping:
./loop4simviz2.py b 0 2 0.25 -yaxis -0.5 0.5 -A 4
|

Remarks
 | Reports:
tmp_c.gif # animated GIF (movie)
animate tmp_c.gif
tmp_c_runs.html # browsable HTML document
|
 | All experiments are archived in a directory with a filename reflecting the varying parameter:
tmp_m_2.1 tmp_b_0 tmp_c_29
|
 | All generated files/directories start with tmp so it is easy to clean up hundreds of experiments
|
 | Try the listed loop4simviz2.py commands!!
|

Exercise
 | Make a summary report with the equation, a picture of the system, the command-line arguments, and a movie of the solution
|
 | Make a link to a detailed report with plots of all the individual experiments
|
 | Demo:
./loop4simviz2_2html.py m 0.1 6.1 0.5 -yaxis -0.5 0.5 \
-noscreenplot
ls -d tmp_*
firefox tmp_m_summary.html
|

Increased quality of scientific work
 | Archiving of experiments and having a system for uniquely relating input data to visualizations or result files are fundamental for reliable scientific investigations
|
 | The experiments can easily be reproduced
|
 | New (large) sets of experiments can be generated
|
 | All these items contribute to increased quality and reliability of computer experiments
|

New example: converting data file formats
 | Input file with time series data:
some comment line
1.5
measurements model1 model2
0.0 0.1 1.0
0.1 0.1 0.188
0.2 0.2 0.25
Contents: comment line, time step, headings, time series data
|
 | Goal: split file into two-column files, one for each time series
|
 | Script: interpret input file, split text, extract data and write files
|

Example on an output file
 | The model1.dat file, arising from column no 2,
becomes
0 0.1
1.5 0.1
3 0.2
|
 | The time step parameter, here 1.5, is used to generate the
first column
|

Program flow
 | Read inputfile name (1st command-line arg.)
|
 | Open input file
|
 | Read and skip the 1st (comment) line
|
 | Extract time step from the 2nd line
|
 | Read time series names from the 3rd line
|
 | Make a list of file objects, one for each time series
|
 | Read the rest of the file, line by line:
 | split lines into y values
|  | write t and y value to file, for all series
|
|
File: src/py/intro/convert1.py

What to learn
 | Reading and writing files
|
 | Sublists
|
 | List of file objects
|
 | Dictionaries
|
 | Arrays of numbers
|
 | List comprehension
|
 | Refactoring a flat script as functions in a module
|

Reading in the first 3 lines
 | Open file and read comment line:
infilename = sys.argv[1]
ifile = open(infilename, 'r') # open for reading
line = ifile.readline()
|
 | Read time step from the next line:
dt = float(ifile.readline())
|
 | Read next line containing the curvenames:
ynames = ifile.readline().split()
|

Output to many files
 |
Make a list of file objects for output of each time series:
outfiles = []
for name in ynames:
outfiles.append(open(name + '.dat', 'w'))
|

Writing output
 |
Read each line, split into y values, write to output files:
t = 0.0 # t value
# read the rest of the file line by line:
while 1:
line = ifile.readline()
if not line: break
yvalues = line.split()
# skip blank lines:
if len(yvalues) == 0: continue
for i in range(len(outfiles)):
outfiles[i].write('%12g %12.5e\n' % \
(t, float(yvalues[i])))
t += dt
for file in outfiles:
file.close()
|

Dictionaries
 | Dictionary = array with a text as index
|
 | Also called hash or associative array in other languages
|
 | Can store 'anything':
prm['damping'] = 0.2 # number
def x3(x):
return x*x*x
prm['stiffness'] = x3 # function object
prm['model1'] = [1.2, 1.5, 0.1] # list object
|
 | The text index is called key
|

Dictionaries for our application
 |
Could store the time series in memory as a dictionary of lists; the list items are the y values and the y names are the keys
y = {} # declare empty dictionary
# ynames: names of y curves
for name in ynames:
y[name] = [] # for each key, make empty list
lines = ifile.readlines() # list of all lines
...
for line in lines[3:]:
yvalues = [float(x) for x in line.split()]
i = 0 # counter for yvalues
for name in ynames:
y[name].append(yvalues[i]); i += 1
|
File: src/py/intro/convert2.py

Dissection of the previous slide
 | Specifying a sublist, e.g., the 4th line until the last line: lines[3:]
Transforming all words in a line to floats:
yvalues = [float(x) for x in line.split()]
# same as
numbers = line.split()
yvalues = []
for s in numbers:
yvalues.append(float(s))
|

The items in a dictionary
 | The input file
some comment line
1.5
measurements model1 model2
0.0 0.1 1.0
0.1 0.1 0.188
0.2 0.2 0.25
results in the following y dictionary:
'measurements': [0.0, 0.1, 0.2],
'model1': [0.1, 0.1, 0.2],
'model2': [1.0, 0.188, 0.25]
(this output is plain print: print y)
|

Remarks
 | Fortran/C programmers tend to think of indices as integers
|
 | Scripters make heavy use of dictionaries and text-type indices (keys)
|
 | Python dictionaries can use (almost) any object as key (!)
|
 | A dictionary is also often called hash (e.g. in Perl) or associative array
|
 | Examples will demonstrate their use
|

Next step: make the script reusable
 | The previous script is ``flat''
(start at top, run to bottom)
|
 | Parts of it may be reusable
|
 | We may like to load data from file, operate on data, and then dump data
|
 | Let's refactor the script:
 | make a load data function
|  | make a dump data function
|  | collect these two functions in a reusable module
|
|

The load data function
def load_data(filename):
f = open(filename, 'r'); lines = f.readlines(); f.close()
dt = float(lines[1])
ynames = lines[2].split()
y = {}
for name in ynames: # make y a dictionary of (empty) lists
y[name] = []
for line in lines[3:]:
yvalues = [float(yi) for yi in line.split()]
if len(yvalues) == 0: continue # skip blank lines
for name, value in zip(ynames, yvalues):
y[name].append(value)
return y, dt

How to call the load data function
 | Note: the function returns two (!) values;
a dictionary of lists, plus a float
|
 | It is common that output data from a Python function are returned, and multiple data structures can be returned (actually packed as a tuple, a kind of ``constant list'')
|
 | Here is how the function is called:
y, dt = load_data('somedatafile.dat')
print y
Output from print y:
>>> y
{'tmp-model2': [1.0, 0.188, 0.25],
'tmp-model1': [0.10000000000000001, 0.10000000000000001,
0.20000000000000001],
'tmp-measurements': [0.0, 0.10000000000000001,
0.20000000000000001]}
|

Iterating over several lists
 | C/C++/Java/Fortran-like iteration over two arrays/lists:
for i in range(len(list)):
e1 = list1[i]; e2 = list2[i]
# work with e1 and e2
|
 | Pythonic version:
for e1, e2 in zip(list1, list2):
# work with element e1 from list1 and e2 from list2
For example,
for name, value in zip(ynames, yvalues):
y[name].append(value)
|

The dump data function
def dump_data(y, dt):
# write out 2-column files with t and y[name] for each name:
for name in y.keys():
ofile = open(name+'.dat', 'w')
for k in range(len(y[name])):
ofile.write('%12g %12.5e\n' % (k*dt, y[name][k]))
ofile.close()

Reusing the functions
 | Our goal is to reuse load_data and dump_data, possibly with some operations on y in between:
from convert3 import load_data, dump_data
y, timestep = load_data('.convert_infile1')
from math import fabs
for name in y: # run through keys in y
maxabsy = max([fabs(yval) for yval in y[name]])
print 'max abs(y[%s](t)) = %g' % (name, maxabsy)
dump_data(y, timestep)
|
 | Then we need to make a module convert3!
|

How to make a module
 | Collect the functions in the module in a file, here the file is called convert3.py
|
 | We have then made a module convert3
|
 | The usage is as exemplified on the previous slide
|

Module with application script
 | The scripts convert1.py and convert2.py load and dump data - this functionality can be reproduced by an application script using convert3
|
 | The application script can be included in the module:
if __name__ == '__main__':
import sys
try:
infilename = sys.argv[1]
except:
usage = 'Usage: %s infile' % sys.argv[0]
print usage; sys.exit(1)
y, dt = load_data(infilename)
dump_data(y, dt)
|
 | If the module file is run as a script, the if test is true and the application script is run
|
 | If the module is imported in a script, the if test is false and no statements are executed
|

Usage of convert3.py
 | As script:
unix> ./convert3.py someinputfile.dat
|
 | As module:
import convert3
y, dt = convert3.load_data('someinputfile.dat')
# do more with y?
dump_data(y, dt)
|
 | The application script at the end also serves as an example on
how to use the module
|

How to solve exercises
 | Construct an example on the functionality of the script, if that is not included in the problem description
|
 | Write very high-level pseudo code with words
|
 | Scan known examples for constructions and functionality that can come into use
|
 | Look up man pages, reference manuals, FAQs, or textbooks for functionality you have minor familiarity with, or to clarify syntax details
|
 | Search the Internet if the documentation from the latter point does not provide sufficient answers
|

Example: write a join function
 | Exercise:
Write a function myjoin that concatenates a list of strings to a single string, with a specified delimiter between the list elements. That is, myjoin is supposed to be an implementation of a string's join method in terms of basic string operations.
|
 | Functionality:
s = myjoin(['s1', 's2', 's3'], '*')
# s becomes 's1*s2*s3'
|

The next steps
 | Pseudo code:
function myjoin(list, delimiter)
joined = first element in list
for element in rest of list:
concatenate joined, delimiter and element
return joined
|
 | Known examples: string concatenation (+ operator) from hw.py, list indexing (list[0]) from datatrans1.py, sublist extraction (list[1:]) from convert1.py, function construction from datatrans1.py
|

Refined pseudo code
def myjoin(list, delimiter):
joined = list[0]
for element in list[1:]:
joined += delimiter + element
return joined
That's it!

How to present the answer to an exercise
 | Use comments to explain ideas
|
 | Use descriptive variable names to reduce the need for more comments
|
 | Find generic solutions (unless the code size explodes)
|
 | Strive at compact code, but not too compact
|
 | Always construct a demonstrating running example and include in it
the source code file inside triple-quoted strings:
"""
unix> python hw.py 3.1459
Hello, World! sin(3.1459)=-0.00430733309102
"""
|
 | Invoke the Python interpreter and run import this
|

How to print exercises with a2ps
 | Here is a suitable command for printing exercises:
Unix> a2ps --line-numbers=1 -4 -o outputfile.ps *.py
This prints all *.py files, with 4 (because of -4) pages per sheet
|
 | See man a2ps for more info about this command
|

Array computing and visualization

Contents
 | Efficient array computing in Python
|
 | Creating arrays
|
 | Indexing/slicing arrays
|
 | Random numbers
|
 | Linear algebra
|
 | Plotting
|

More info
 | Ch. 4 in the course book
|
 | www.scipy.org
|
 | The NumPy manual
|
 | The SciPy tutorial
|

Numerical Python (NumPy)
 | NumPy enables efficient numerical computing in Python
|
 | NumPy is a package of modules, which offers efficient arrays (contiguous storage) with associated array operations coded in C or Fortran
|
 | There are three implementations of Numerical Python
 | Numeric from the mid 90s (still widely used)
|  | numarray from about 2000
|  | numpy from 2006
|
|
 | We recommend to use numpy (by Travis Oliphant)
from numpy import *
|

A taste of NumPy: a least-squares procedure
x = linspace(0.0, 1.0, n) # coordinates
y_line = -2*x + 3
y = y_line + random.normal(0, 0.25, n) # line with noise
# goal: fit a line to the data points x, y
# create and solve least squares system:
A = array([x, ones(n)])
A = A.transpose()
result = linalg.lstsq(A, y)
# result is a 4-tuple, the solution (a,b) is the 1st entry:
a, b = result[0]
plot(x, y, 'o', # data points w/noise
x, y_line, 'r', # original line
x, a*x + b, 'b') # fitted lines
legend('data points', 'original line', 'fitted line')
hardcopy('myplot.png')

Resulting plot
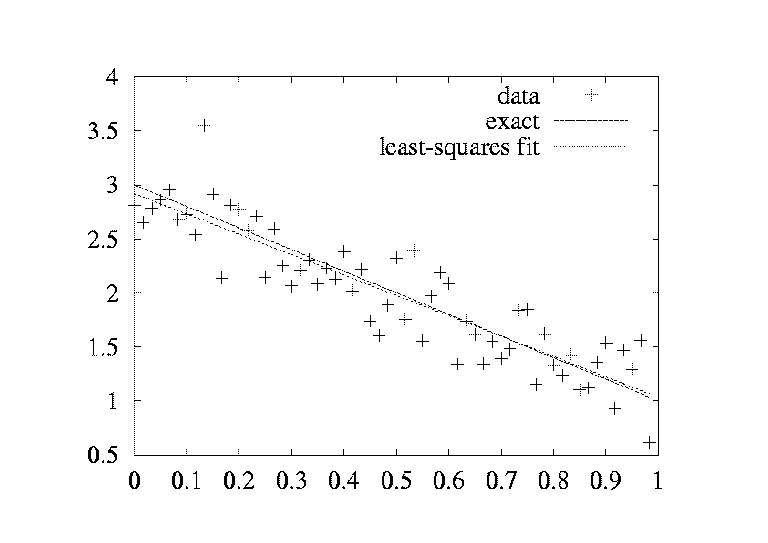

Making arrays
>>> from numpy import *
>>> n = 4
>>> a = zeros(n) # one-dim. array of length n
>>> print a
[ 0. 0. 0. 0.]
>>> a
array([ 0., 0., 0., 0.])
>>> p = q = 2
>>> a = zeros((p,q,3)) # p*q*3 three-dim. array
>>> print a
[[[ 0. 0. 0.]
[ 0. 0. 0.]]
[[ 0. 0. 0.]
[ 0. 0. 0.]]]
>>> a.shape # a's dimension
(2, 2, 3)

Making float, int, complex arrays
>>> a = zeros(3)
>>> print a.dtype # a's data type
float64
>>> a = zeros(3, int)
>>> print a
[0 0 0]
>>> print a.dtype
int32
>>> a = zeros(3, float32) # single precision
>>> print a
[ 0. 0. 0.]
>>> print a.dtype
float32
>>> a = zeros(3, complex)
>>> a
array([ 0.+0.j, 0.+0.j, 0.+0.j])
>>> a.dtype
dtype('complex128')
>>> given an array a, make a new array of same dimension
>>> and data type:
>>> x = zeros(a.shape, a.dtype)

Array with a sequence of numbers
 | linspace(a, b, n) generates n uniformly spaced
coordinates, starting with a and ending with b
>>> x = linspace(-5, 5, 11)
>>> print x
[-5. -4. -3. -2. -1. 0. 1. 2. 3. 4. 5.]
|
 | A special compact syntax is also available:
>>> a = r_[-5:5:11j] # same as linspace(-5, 5, 11)
>>> print a
[-5. -4. -3. -2. -1. 0. 1. 2. 3. 4. 5.]
|
 | arange works like range (xrange)
>>> x = arange(-5, 5, 1, float)
>>> print x # upper limit 5 is not included!!
[-5. -4. -3. -2. -1. 0. 1. 2. 3. 4.]
|

Warning: arange is dangerous
 | arange's upper limit may or may not be included
(due to round-off errors)
|
 | Better to use a safer method: seq(start, stop, increment)
>>> from scitools.numpyutils import seq
>>> x = seq(-5, 5, 1)
>>> print x # upper limit always included
[-5. -4. -3. -2. -1. 0. 1. 2. 3. 4. 5.]
|

Array construction from a Python list
| array(list, [datatype]) generates an array from a list:
>>> pl = [0, 1.2, 4, -9.1, 5, 8]
>>> a = array(pl)
|
 | The array elements are of the simplest possible type:
>>> z = array([1, 2, 3])
>>> print z # array of integers
[1 2 3]
>>> z = array([1, 2, 3], float)
>>> print z
[ 1. 2. 3.]
|
 | A two-dim. array from two one-dim. lists:
>>> x = [0, 0.5, 1]; y = [-6.1, -2, 1.2] # Python lists
>>> a = array([x, y]) # form array with x and y as rows
|
 | From array to list: alist = a.tolist()
|

From ``anything'' to a NumPy array
 | Given an object a,
a = asarray(a)
converts a to a NumPy array (if possible/necessary)
|
 | Arrays can be ordered as in C (default) or Fortran:
a = asarray(a, order='Fortran')
isfortran(a) # returns True if a's order is Fortran
|
 | Use asarray to, e.g., allow flexible arguments in functions:
def myfunc(some_sequence):
a = asarray(some_sequence)
return 3*a - 5
myfunc([1,2,3]) # list argument
myfunc((-1,1)) # tuple argument
myfunc(zeros(10)) # array argument
myfunc(-4.5) # float argument
myfunc(6) # int argument
|

Changing array dimensions
>>> a = array([0, 1.2, 4, -9.1, 5, 8])
>>> a.shape = (2,3) # turn a into a 2x3 matrix
>>> print a
[[ 0. 1.2 4. ]
[-9.1 5. 8. ]]
>>> a.size
6
>>> a.shape = (a.size,) # turn a into a vector of length 6 again
>>> a.shape
(6,)
>>> print a
[ 0. 1.2 4. -9.1 5. 8. ]
>>> a = a.reshape(2,3) # same effect as setting a.shape
>>> a.shape
(2, 3)

Array initialization from a Python function
>>> def myfunc(i, j):
... return (i+1)*(j+4-i)
...
>>> # make 3x6 array where a[i,j] = myfunc(i,j):
>>> a = fromfunction(myfunc, (3,6))
>>> a
array([[ 4., 5., 6., 7., 8., 9.],
[ 6., 8., 10., 12., 14., 16.],
[ 6., 9., 12., 15., 18., 21.]])

Basic array indexing
Note: all integer indices in Python start at 0!
a = linspace(-1, 1, 6)
a[2:4] = -1 # set a[2] and a[3] equal to -1
a[-1] = a[0] # set last element equal to first one
a[:] = 0 # set all elements of a equal to 0
a.fill(0) # set all elements of a equal to 0
a.shape = (2,3) # turn a into a 2x3 matrix
print a[0,1] # print element (0,1)
a[i,j] = 10 # assignment to element (i,j)
a[i][j] = 10 # equivalent syntax (slower)
print a[:,k] # print column with index k
print a[1,:] # print second row
a[:,:] = 0 # set all elements of a equal to 0

More advanced array indexing
>>> a = linspace(0, 29, 30)
>>> a.shape = (5,6)
>>> a
array([[ 0., 1., 2., 3., 4., 5.,]
[ 6., 7., 8., 9., 10., 11.,]
[ 12., 13., 14., 15., 16., 17.,]
[ 18., 19., 20., 21., 22., 23.,]
[ 24., 25., 26., 27., 28., 29.,]])
>>> a[1:3,:-1:2] # a[i,j] for i=1,2 and j=0,2,4
array([[ 6., 8., 10.],
[ 12., 14., 16.]])
>>> a[::3,2:-1:2] # a[i,j] for i=0,3 and j=2,4
array([[ 2., 4.],
[ 20., 22.]])
>>> i = slice(None, None, 3); j = slice(2, -1, 2)
>>> a[i,j]
array([[ 2., 4.],
[ 20., 22.]])

Slices refer the array data
 | With a as list, a[:] makes a copy of the data
|
 | With a as array, a[:] is a reference to the data
>>> b = a[1,:] # extract 2nd row of a
>>> print a[1,1]
12.0
>>> b[1] = 2
>>> print a[1,1]
2.0 # change in b is reflected in a!
|
 | Take a copy to avoid referencing via slices:
>>> b = a[1,:].copy()
>>> print a[1,1]
12.0
>>> b[1] = 2 # b and a are two different arrays now
>>> print a[1,1]
12.0 # a is not affected by change in b
|

Loops over arrays (1)
 | Standard loop over each element:
for i in xrange(a.shape[0]):
for j in xrange(a.shape[1]):
a[i,j] = (i+1)*(j+1)*(j+2)
print 'a[%d,%d]=%g ' % (i,j,a[i,j]),
print # newline after each row
|
 | A standard for loop iterates over the first index:
>>> print a
[[ 2. 6. 12.]
[ 4. 12. 24.]]
>>> for e in a:
... print e
...
[ 2. 6. 12.]
[ 4. 12. 24.]
|

Loops over arrays (2)
 | View array as one-dimensional and iterate over all elements:
for e in a.ravel():
print e
Use ravel() only when reading elements, for assigning it is better to use shape or reshape first!
|
 | For loop over all index tuples and values:
>>> for index, value in ndenumerate(a):
... print index, value
...
(0, 0) 2.0
(0, 1) 6.0
(0, 2) 12.0
(1, 0) 4.0
(1, 1) 12.0
(1, 2) 24.0
|

Array computations
 | Arithmetic operations can be used with arrays:
b = 3*a - 1 # a is array, b becomes array
1) compute t1 = 3*a, 2) compute t2= t1 - 1, 3) set b = t2
|
 | Array operations are much faster than element-wise operations:
>>> import time # module for measuring CPU time
>>> a = linspace(0, 1, 1E+07) # create some array
>>> t0 = time.clock()
>>> b = 3*a -1
>>> t1 = time.clock() # t1-t0 is the CPU time of 3*a-1
>>> for i in xrange(a.size): b[i] = 3*a[i] - 1
>>> t2 = time.clock()
>>> print '3*a-1: %g sec, loop: %g sec' % (t1-t0, t2-t1)
3*a-1: 2.09 sec, loop: 31.27 sec
|

Standard math functions can take array arguments
# let b be an array
c = sin(b)
c = arcsin(c)
c = sinh(b)
# same functions for the cos and tan families
c = b**2.5 # power function
c = log(b)
c = exp(b)
c = sqrt(b)

Other useful array operations
# a is an array
a.clip(min=3, max=12) # clip elements
a.mean(); mean(a) # mean value
a.var(); var(a) # variance
a.std(); std(a) # standard deviation
median(a)
cov(x,y) # covariance
trapz(a) # Trapezoidal integration
diff(a) # finite differences (da/dx)
# more Matlab-like functions:
corrcoeff, cumprod, diag, eig, eye, fliplr, flipud, max, min,
prod, ptp, rot90, squeeze, sum, svd, tri, tril, triu

More useful array methods and attributes
>>> a = zeros(4) + 3
>>> a
array([ 3., 3., 3., 3.]) # float data
>>> a.item(2) # more efficient than a[2]
3.0
>>> a.itemset(3,-4.5) # more efficient than a[3]=-4.5
>>> a
array([ 3. , 3. , 3. , -4.5])
>>> a.shape = (2,2)
>>> a
array([[ 3. , 3. ],
[ 3. , -4.5]])
>>> a.ravel() # from multi-dim to one-dim
array([ 3. , 3. , 3. , -4.5])
>>> a.ndim # no of dimensions
2
>>> len(a.shape) # no of dimensions
2
>>> rank(a) # no of dimensions
2
>>> a.size # total no of elements
4
>>> b = a.astype(int) # change data type
>>> b
array([3, 3, 3, 3])

Modules for curve plotting and 2D/3D visualization
 | Matplotlib (curve plotting, 2D scalar and vector fields)
|
 | PyX (PostScript/TeX-like drawing)
|
 | Interface to Gnuplot
|
 | Interface to Vtk
|
 | Interface to OpenDX
|
 | Interface to IDL
|
 | Interface to Grace
|
 | Interface to Matlab
|
 | Interface to R
|
 | Interface to Blender
|

Curve plotting with Easyviz
 | Easyviz is a light-weight interface to many plotting packages, using
a Matlab-like syntax
|
 | Goal: write your program using Easyviz (``Matlab'') syntax and postpone your choice of plotting package
|
 | Note: some powerful plotting packages (Vtk, R, matplotlib, ...) may be
troublesome to install, while Gnuplot is easily installed on all platforms
|
 | Easyviz supports (only) the most common plotting commands
|
 | Easyviz is part of SciTools (Simula development)
from scitools.all import *
(imports all of numpy, all of easyviz, plus scitools)
|

Basic Easyviz example
from scitools.all import * # import numpy and plotting
t = linspace(0, 3, 51) # 51 points between 0 and 3
y = t**2*exp(-t**2) # vectorized expression
plot(t, y)
hardcopy('tmp1.eps') # make PostScript image for reports
hardcopy('tmp1.png') # make PNG image for web pages

Decorating the plot
plot(t, y)
xlabel('t')
ylabel('y')
legend('t^2*exp(-t^2)')
axis([0, 3, -0.05, 0.6]) # [tmin, tmax, ymin, ymax]
title('My First Easyviz Demo')
# or
plot(t, y, xlabel='t', ylabel='y',
legend='t^2*exp(-t^2)',
axis=[0, 3, -0.05, 0.6],
title='My First Easyviz Demo',
hardcopy='tmp1.eps',
show=True) # display on the screen (default)

The resulting plot

Plotting several curves in one plot
from scitools.all import * # for curve plotting
def f1(t):
return t**2*exp(-t**2)
def f2(t):
return t**2*f1(t)
t = linspace(0, 3, 51)
y1 = f1(t)
y2 = f2(t)
plot(t, y1)
hold('on') # continue plotting in the same plot
plot(t, y2)
xlabel('t')
ylabel('y')
legend('t^2*exp(-t^2)', 't^4*exp(-t^2)')
title('Plotting two curves in the same plot')
hardcopy('tmp2.eps')

The resulting plot

Example: plot a function given on the command line
| Specify $f(x)$ and $x$ interval as text on the command line:
Unix/DOS> python plotf.py "exp(-0.2*x)*sin(2*pi*x)" 0 4*pi
|
 | Program:
from scitools.all import *
formula = sys.argv[1]
xmin = eval(sys.argv[2])
xmax = eval(sys.argv[3])
x = linspace(xmin, xmax, 101)
y = eval(formula)
plot(x, y, title=formula)
|
 | Thanks to eval, input (text) with correct Python syntax
can be turned to running code on the fly
|

Plotting 2D scalar fields
from scitools.all import *
x = y = linspace(-5, 5, 21)
xv, yv = ndgrid(x, y)
values = sin(sqrt(xv**2 + yv**2))
surf(xv, yv, values)

Adding plot features
# Matlab style commands:
setp(interactive=False)
surf(xv, yv, values)
shading('flat')
colorbar()
colormap(hot())
axis([-6,6,-6,6,-1.5,1.5])
view(35,45)
show()
# Optional Easyviz (Pythonic) short cut:
surf(xv, yv, values,
shading='flat',
colorbar='on',
colormap=hot(),
axis=[-6,6,-6,6,-1.5,1.5],
view=[35,45])

The resulting plot

Other commands for visualizing 2D scalar fields
 | contour (standard contours)), contourf (filled contours), contour3 (elevated contours)
|
 | mesh (elevated mesh),
meshc (elevated mesh with contours in the xy plane)
|
 | surf (colored surface),
surfc (colored surface with contours in the xy plane)
|
 | pcolor (colored cells in a 2D mesh)
|

Commands for visualizing 3D fields
Scalar fields:
 | isosurface
|
 | slice_ (colors in slice plane),
contourslice (contours in slice plane)
|
Vector fields:
 | quiver3 (arrows), (quiver for 2D vector fields)
|
 | streamline, streamtube, streamribbon (flow sheets)
|

More info about Easyviz
 | A plain text version of the Easyviz manual:
pydoc scitools.easyviz
|
 | The HTML version:
http://folk.uio.no/hpl/easyviz/
|
 | Download SciTools (incl.~Easyviz):
http://code.google.com/p/scitools/
|

More about array computing

Integer arrays as indices
 | An integer array or list can be used as (vectorized) index
>>> a = linspace(1, 8, 8)
>>> a
array([ 1., 2., 3., 4., 5., 6., 7., 8.])
>>> a[[1,6,7]] = 10
>>> a
array([ 1., 10., 3., 4., 5., 6., 10., 10.])
>>> a[range(2,8,3)] = -2
>>> a
array([ 1., 10., -2., 4., 5., -2., 10., 10.])
>>> a[a < 0] # pick out the negative elements of a
array([-2., -2.])
>>> a[a < 0] = a.max()
>>> a
array([ 1., 10., 10., 4., 5., 10., 10., 10.])
|
 | Such array indices are important for efficient vectorized code
|

More about references to data
>>> A = array([[1,2,3],[4,5,6]], float)
>>> print A
[[ 1. 2. 3.]
[ 4. 5. 6.]]
>>> b = A[:,1:]
>>> print b
[[ 2. 3.]
[ 5. 6.]]
>>> c = 3*b
>>> b[:,:] = c # this affects A!
>>> print A
[[ 1. 6. 9.]
[ 4. 15. 18.]]
>>> b = 2*c # b refers to new array
>>> b[0,0] = -1 # does not affect A
>>> print A[0,0]
1.0
>>> A[:,:-1] = 3*c # does not affect b
>>> print A
[[ 18. 27. 9.]
[ 45. 54. 18.]]

Complex number computing
>>> from math import sqrt
>>> sqrt(-1)
Traceback (most recent call last):
File "<stdin>", line 1, in <module>
ValueError: math domain error
>>> from numpy import sqrt
>>> sqrt(-1)
Warning: invalid value encountered in sqrt
nan
>>> from cmath import sqrt # complex math functions
>>> sqrt(-1)
1j
>>> sqrt(4) # cmath functions always return complex...
(2+0j)
>>> from numpy.lib.scimath import sqrt
>>> sqrt(4)
2.0 # real when possible
>>> sqrt(-1)
1j # otherwise complex

A root function
# Goal: compute roots of a parabola, return real when possible,
# otherwise complex
def roots(a, b, c):
# compute roots of a*x^2 + b*x + c = 0
from numpy.lib.scimath import sqrt
q = sqrt(b**2 - 4*a*c) # q is real or complex
r1 = (-b + q)/(2*a)
r2 = (-b - q)/(2*a)
return r1, r2
>>> a = 1; b = 2; c = 100
>>> roots(a, b, c) # complex roots
((-1+9.94987437107j), (-1-9.94987437107j))
>>> a = 1; b = 4; c = 1
>>> roots(a, b, c) # real roots
(-0.267949192431, -3.73205080757)

Array type and data type
>>> import numpy
>>> a = numpy.zeros(5)
>>> type(a)
<type 'numpy.ndarray'>
>>> isinstance(a, ndarray) # is a of type ndarray?
True
>>> a.dtype # data (element) type object
dtype('float64')
>>> a.dtype.name
'float64'
>>> a.dtype.char # character code
'd'
>>> a.dtype.itemsize # no of bytes per array element
8
>>> b = zeros(6, float32)
>>> a.dtype == b.dtype # do a and b have the same data type?
False
>>> c = zeros(2, float)
>>> a.dtype == c.dtype
True

Matrix objects (1)
 | NumPy has an array type, matrix, much like Matlab's array type
>>> x1 = array([1, 2, 3], float)
>>> x2 = matrix(x1) # or just mat(x)
>>> x2 # row vector
matrix([[ 1., 2., 3.]])
>>> x3 = matrix(x1.transpose() # column vector
>>> x3
matrix([[ 1.],
[ 2.],
[ 3.]])
>>> type(x3)
<class 'numpy.core.defmatrix.matrix'>
>>> isinstance(x3, matrix)
True
|
 | Only 1- and 2-dimensional arrays can be matrix
|

Matrix objects (2)
 | For matrix objects, the * operator means matrix-matrix or matrix-vector multiplication (not elementwise multiplication)
>>> A = eye(3) # identity matrix
>>> A = mat(A) # turn array to matrix
>>> A
matrix([[ 1., 0., 0.],
[ 0., 1., 0.],
[ 0., 0., 1.]])
>>> y2 = x2*A # vector-matrix product
>>> y2
matrix([[ 1., 2., 3.]])
>>> y3 = A*x3 # matrix-vector product
>>> y3
matrix([[ 1.],
[ 2.],
[ 3.]])
|

Compound expressions generate temporary arrays
 | Let us evaluate f1(x) for a vector x:
def f1(x):
return exp(-x*x)*log(1+x*sin(x))
|
 | Calling f1(x) is equivalent to the code
temp1 = -x
temp2 = temp1*x
temp3 = exp(temp2)
temp4 = sin(x)}
temp5 = x*temp4
temp6 = 1 + temp4
temp7 = log(temp5)
result = temp3*temp7
|

In-place array arithmetics
 | Expressions like 3*a-1 generates temporary arrays
|
 | With in-place modifications of arrays, we can avoid temporary arrays
(to some extent)
b = a
b *= 3 # or multiply(b, 3, b)
b -= 1 # or subtract(b, 1, b)
Note: a is changed, use b = a.copy()
|
 | In-place operations:
a *= 3.0 # multiply a's elements by 3
a -= 1.0 # subtract 1 from each element
a /= 3.0 # divide each element by 3
a += 1.0 # add 1 to each element
a **= 2.0 # square all elements
|
 | Assign values to all elements of an existing array:
a[:] = 3*c - 1 # insert values into a
a = 3*c - 1 # let a refer to new array object
|

Vectorization (1)
 | Loops over an array run slowly
|
 | Vectorization = replace explicit loops by functions calls such that the whole loop is implemented in C (or Fortran)
|
 | Explicit loops:
r = zeros(x.shape, x.dtype)
for i in xrange(x.size):
r[i] = sin(x[i])
|
 | Vectorized version:
r = sin(x)
|
 | Arithmetic expressions work for both scalars and arrays
|
 | Many fundamental functions work for scalars and arrays
|
 | Ex: x**2 + abs(x) works for x scalar or array
|

Vectorization (2)
A mathematical
function written for scalar arguments can (normally) take array arguments:
>>> def f(x):
... return x**2 + sinh(x)*exp(-x) + 1
...
>>> # scalar argument:
>>> x = 2
>>> f(x)
5.4908421805556333
>>> # array argument:
>>> y = array([2, -1, 0, 1.5])
>>> f(y)
array([ 5.49084218, -1.19452805, 1. , 3.72510647])

Vectorization of functions with if tests; problem
 | Consider a function with an if test:
def somefunc(x):
if x < 0:
return 0
else:
return sin(x)
# or
def somefunc(x): return 0 if x < 0 else sin(x)
|
 | This function works with a scalar x but not an array
|
 | Problem: x<0 results in a boolean array, not a boolean value
that can be used in the if test
>>> x = linspace(-1, 1, 3); print x
[-1. 0. 1.]
>>> y = x < 0
>>> y
array([ True, False, False], dtype=bool)
>>> bool(y) # turn object into a scalar boolean value
...
ValueError: The truth value of an array with more than one
element is ambiguous. Use a.any() or a.all()
|

Vectorization of functions with if tests; solutions
 | Simplest remedy: use NumPy's vectorize class to allow
array arguments to a function:
>>> somefuncv = vectorize(somefunc, otypes='d')
>>> # test:
>>> x = linspace(-1, 1, 3); print x
[-1. 0. 1.]
>>> somefuncv(x)
array([ 0. , 0. , 0.84147098])
Note: The data type must be specified as a character ('d' for double)
|
 | The speed of somefuncv is unfortunately quite slow
|
 | A better solution, using where:
def somefuncv2(x):
x2 = sin(x)
return where(x < 0, 0, x2)
|

General vectorization of if-else tests
def f(x): # scalar x
if condition:
x = <expression1>
else:
x = <expression2>
return x
def f_vectorized(x): # scalar or array x
x1 = <expression1>
x2 = <expression2>
return where(condition, x1, x2)

Vectorization via slicing
 | Consider a recursion scheme like
\]
(which arises from a one-dimensional diffusion equation)
|
 | Straightforward (slow) Python implementation:
n = size(u)-1
for i in xrange(1,n,1):
u_new[i] = beta*u[i-1] + (1-2*beta)*u[i] + beta*u[i+1]
|
 | Slices enable us to vectorize the expression:
u[1:n] = beta*u[0:n-1] + (1-2*beta)*u[1:n] + beta*u[2:n+1]
|
 | Speed-up: factor 10--150 (150 for 3D arrays)
|

Random numbers
 | Drawing scalar random numbers:
import random
random.seed(2198) # control the seed
u = random.random() # uniform number on [0,1)
u = random.uniform(-1, 1) # uniform number on [-1,1)
u = random.gauss(m, s) # number from N(m,s)
|
 | Vectorized drawing of random numbers (arrays):
from numpy import random
random.seed(12) # set seed
u = random.random(n) # n uniform numbers on (0,1)
u = random.uniform(-1, 1, n) # n uniform numbers on (-1,1)
u = random.normal(m, s, n) # n numbers from N(m,s)
|
 | Note that both modules have the name random! A remedy:
import random as random_number # rename random for scalars
from numpy import * # random is now numpy.random
|

Basic linear algebra
NumPy contains the linalg module for
 | solving linear systems
|
 | computing the determinant of a matrix
|
 | computing the inverse of a matrix
|
 | computing eigenvalues and eigenvectors of a matrix
|
 | solving least-squares problems
|
 | computing the singular value decomposition of a matrix
|
 | computing the Cholesky decomposition of a matrix
|

A linear algebra session
from numpy import * # includes import of linalg
# fill matrix A and vectors x and b
b = dot(A, x) # matrix-vector product
y = linalg.solve(A, b) # solve A*y = b
if allclose(x, y, atol=1.0E-12, rtol=1.0E-12):
print 'correct solution!'
d = linalg.det(A)
B = linalg.inv(A)
# check result:
R = dot(A, B) - eye(n) # residual
R_norm = linalg.norm(R) # Frobenius norm of matrix R
print 'Residual R = A*A-inverse - I:', R_norm
A_eigenvalues = linalg.eigvals(A) # eigenvalues only
A_eigenvalues, A_eigenvectors = linalg.eig(A)
for e, v in zip(A_eigenvalues, A_eigenvectors):
print 'eigenvalue %g has corresponding vector\n%s' % (e, v)

A least-squares procedure
x = linspace(0.0, 1.0, n) # coordinates
y_line = -2*x + 3
y = y_line + random.normal(0, 0.25, n) # line with noise
# goal: fit a line to the data points x, y
# create and solve least squares system:
A = array([x, ones(n)])
A = A.transpose()
result = linalg.lstsq(A, y)
# result is a 4-tuple, the solution (a,b) is the 1st entry:
a, b = result[0]
plot(x, y, 'o', # data points w/noise
x, y_line, 'r', # original line
x, a*x + b, 'b') # fitted lines
legend('data points', 'original line', 'fitted line')
hardcopy('myplot.png')

File I/O with arrays; plain ASCII format
| Plain text output to file (just dump repr(array)):
a = linspace(1, 21, 21); a.shape = (2,10)
file = open('tmp.dat', 'w')
file.write('Here is an array a:\n')
file.write(repr(a)) # dump string representation of a
file.close()
|
| Plain text input (just take eval on input line):
file = open('tmp.dat', 'r')
file.readline() # load the first line (a comment)
b = eval(file.read())
file.close()
|

File I/O with arrays; binary pickling
 | Dump arrays with cPickle:
# a1 and a2 are two arrays
import cPickle
file = open('tmp.dat', 'wb')
file.write('This is the array a1:\n')
cPickle.dump(a1, file)
file.write('Here is another array a2:\n')
cPickle.dump(a2, file)
file.close()
|
 | Read in the arrays again (in correct order):
file = open('tmp.dat', 'rb')
file.readline() # swallow the initial comment line
b1 = cPickle.load(file)
file.readline() # swallow next comment line
b2 = cPickle.load(file)
file.close()
|

ScientificPython
 | ScientificPython (by Konrad Hinsen)
|
 | Modules for automatic differentiation,
interpolation, data fitting via nonlinear least-squares, root finding,
numerical integration, basic statistics,
histogram computation, visualization,
parallel computing (via MPI or BSP),
physical quantities with dimension (units),
3D vectors/tensors, polynomials, I/O support for Fortran files and netCDF
|
 | Very easy to install
|

ScientificPython: numbers with units
>>> from Scientific.Physics.PhysicalQuantities \
import PhysicalQuantity as PQ
>>> m = PQ(12, 'kg') # number, dimension
>>> a = PQ('0.88 km/s**2') # alternative syntax (string)
>>> F = m*a
>>> F
PhysicalQuantity(10.56,'kg*km/s**2')
>>> F = F.inBaseUnits()
>>> F
PhysicalQuantity(10560.0,'m*kg/s**2')
>>> F.convertToUnit('MN') # convert to Mega Newton
>>> F
PhysicalQuantity(0.01056,'MN')
>>> F = F + PQ(0.1, 'kPa*m**2') # kilo Pascal m^2
>>> F
PhysicalQuantity(0.010759999999999999,'MN')
>>> F.getValue()
0.010759999999999999

SciPy
 | SciPy is a comprehensive package (by Eric Jones, Travis Oliphant,
Pearu Peterson) for scientific computing with Python
|
 | Much overlap with ScientificPython
|
 | SciPy interfaces many classical Fortran packages from Netlib (QUADPACK, ODEPACK, MINPACK, ...)
|
 | Functionality: special functions, linear algebra, numerical integration, ODEs, random variables and statistics, optimization, root finding, interpolation, ...
|
 | May require some installation efforts (applies ATLAS)
|
 | See www.scipy.org
|

SymPy: symbolic computing in Python
 | SymPy is a Python package for symbolic computing
|
 | Easy to install, easy to extend
|
 | Easy to use:
>>> from sympy import *
>>> x = Symbol('x')
>>> f = cos(acos(x))
>>> f
cos(acos(x))
>>> sin(x).series(x, 4) # 4 terms of the Taylor series
x - 1/6*x**3 + O(x**4)
>>> dcos = diff(cos(2*x), x)
>>> dcos
-2*sin(2*x)
>>> dcos.subs(x, pi).evalf() # x=pi, float evaluation
0
>>> I = integrate(log(x), x)
>>> print I
-x + x*log(x)
|

Python + Matlab = true
 |
A Python module, pymat, enables communication with Matlab:
from numpy import *
import pymat
x = linspace(0, 4*math.pi, 11)
m = pymat.open()
# can send numpy arrays to Matlab:
pymat.put(m, 'x', x);
pymat.eval(m, 'y = sin(x)')
pymat.eval(m, 'plot(x,y)')
# get a new numpy array back:
y = pymat.get(m, 'y')
|

Intro to mixed language programming

Contents
 | Why Python and C are two different worlds
|
 | Wrapper code
|
 | Wrapper tools
|
 | F2PY: wrapping Fortran (and C) code
|
 | SWIG: wrapping C and C++ code
|

More info
 | Ch. 5 in the course book
|
 | F2PY manual
|
 | SWIG manual
|
 | Examples coming with the SWIG source code
|
 | Ch. 9 and 10 in the course book
|

Optimizing slow Python code
 | Identify bottlenecks (via profiling)
|
 | Migrate slow functions to Fortran, C, or C++
|
 | Tools make it easy to combine Python with Fortran, C, or C++
|

Getting started: Scientific Hello World
 | Python-F77 via F2PY
|
 | Python-C via SWIG
|
 | Python-C++ via SWIG
|
Later: Python interface to oscillator code for
interactive computational steering of simulations (using F2PY)

The nature of Python vs. C
 | A Python variable can hold different objects:
d = 3.2 # d holds a float
d = 'txt' # d holds a string
d = Button(frame, text='push') # instance of class Button
|
 | In C, C++ and Fortran, a variable is declared of a specific type:
double d; d = 4.2;
d = "some string"; /* illegal, compiler error */
|
 | This difference makes it quite complicated to call
C, C++ or Fortran from Python
|

Calling C from Python
 | Suppose we have a C function
extern double hw1(double r1, double r2);
|
 | We want to call this from Python as
from hw import hw1
r1 = 1.2; r2 = -1.2
s = hw1(r1, r2)
|
 | The Python variables r1 and r2 hold numbers (float), we need to extract these in the C code, convert to double variables, then call hw1, and finally convert the double result to a Python float
|
 | All this conversion is done in wrapper code
|

Wrapper code
 | Every object in Python is represented by C struct PyObject
|
 | Wrapper code converts between PyObject variables and plain C variables (from PyObject r1 and r2 to double, and
double result to PyObject):
static PyObject *_wrap_hw1(PyObject *self, PyObject *args) {
PyObject *resultobj;
double arg1, arg2, result;
PyArg_ParseTuple(args,(char *)"dd:hw1",&arg1,&arg2))
result = hw1(arg1,arg2);
resultobj = PyFloat_FromDouble(result);
return resultobj;
}
|

Extension modules
 | The wrapper function and hw1 must be compiled and linked to a shared library file
|
 | This file can be loaded in Python as module
|
 | Such modules written in other languages are
called extension modules
|

Writing wrapper code
 | A wrapper function is needed for each C function we want to call from Python
|
 | Wrapper codes are tedious to write
|
 | There are tools for automating wrapper code development
|
 | We shall use SWIG (for C/C++) and F2PY (for Fortran)
|

Integration issues
 | Direct calls through wrapper code enables efficient data transfer; large arrays can be sent by pointers
|
 | COM, CORBA, ILU, .NET are different technologies; more complex, less efficient, but safer (data are copied)
|
 | Jython provides a seamless integration of Python and Java
|

Scientific Hello World example
 | Consider this Scientific Hello World module (hw):
import math
def hw1(r1, r2):
s = math.sin(r1 + r2)
return s
def hw2(r1, r2):
s = math.sin(r1 + r2)
print 'Hello, World! sin(%g+%g)=%g' % (r1,r2,s)
Usage:
from hw import hw1, hw2
print hw1(1.0, 0)
hw2(1.0, 0)
|
 | We want to implement the module in Fortran 77, C and C++, and use it as if it were a pure Python module
|

Fortran 77 implementation
 | We start with Fortran (F77)
|
 | F77 code in a file hw.f:
real*8 function hw1(r1, r2)
real*8 r1, r2
hw1 = sin(r1 + r2)
return
end
subroutine hw2(r1, r2)
real*8 r1, r2, s
s = sin(r1 + r2)
write(*,1000) 'Hello, World! sin(',r1+r2,')=',s
1000 format(A,F6.3,A,F8.6)
return
end
|

One-slide F77 course
 | Fortran is case insensitive (reAL is as good as real)
|
 | One statement per line, must start in column 7 or later
|
 | Comma on separate lines
|
 | All function arguments are input and output
(as pointers in C, or references in C++)
|
 | A function returning one value is called function
|
 | A function returning no value is called subroutine
|
 | Types: real, double precision, real*4, real*8, integer, character (array)
|
 | Arrays: just add dimension, as in
real*8 a(0:m, 0:n)
|
 | Format control of output requires FORMAT statements
|

Using F2PY
 | F2PY automates integration of Python and Fortran
|
 | Say the F77 code is in the file hw.f
|
 | Run F2PY (-m module name, -c for compile+link):
f2py -m hw -c hw.f
|
 | Load module into Python and test:
from hw import hw1, hw2
print hw1(1.0, 0)
hw2(1.0, 0)
|
 | In Python, hw appears as a module with Python code...
|
 | It cannot be simpler!
|

Call by reference issues
 | In Fortran (and C/C++) functions often modify arguments; here the result s is an output argument:
subroutine hw3(r1, r2, s)
real*8 r1, r2, s
s = sin(r1 + r2)
return
end
|
 | Running F2PY results in a module with wrong behavior:
>>> from hw import hw3
>>> r1 = 1; r2 = -1; s = 10
>>> hw3(r1, r2, s)
>>> print s
10 # should be 0
|
 | Why? F2PY assumes that all arguments are input arguments
|
 | Output arguments must be explicitly specified!
|

General adjustment of interfaces to Fortran
 | Function with multiple input and output variables
subroutine somef(i1, i2, o1, o2, o3, o4, io1)
|
 |
input: i1, i2
|
 | output: o1, ..., o4
|
 | input and output: io1
|
 | Pythonic interface, as generated by F2PY:
o1, o2, o3, o4, io1 = somef(i1, i2, io1)
|

Check F2PY-generated doc strings
 | What happened to our hw3 subroutine?
|
 | F2PY generates doc strings that document the interface:
>>> import hw
>>> print hw.__doc__ # brief module doc string
Functions:
hw1 = hw1(r1,r2)
hw2(r1,r2)
hw3(r1,r2,s)
>>> print hw.hw3.__doc__ # more detailed function doc string
hw3 - Function signature:
hw3(r1,r2,s)
Required arguments:
r1 : input float
r2 : input float
s : input float
|
 | We see that hw3 assumes s is input argument!
|
 | Remedy: adjust the interface
|

Interface files
 | We can tailor the interface by editing an F2PY-generated interface file
|
 | Run F2PY in two steps: (i) generate interface file, (ii) generate wrapper code, compile and link
|
 | Generate interface file hw.pyf (-h option):
f2py -m hw -h hw.pyf hw.f
|

Outline of the interface file
 | The interface applies a Fortran 90 module (class) syntax
|
 | Each function/subroutine, its arguments and its return value is specified:
python module hw ! in
interface ! in :hw
...
subroutine hw3(r1,r2,s) ! in :hw:hw.f
real*8 :: r1
real*8 :: r2
real*8 :: s
end subroutine hw3
end interface
end python module hw
(Fortran 90 syntax)
|

Adjustment of the interface
 | We may edit hw.pyf and specify s in hw3 as an output argument, using F90's intent(out) keyword:
python module hw ! in
interface ! in :hw
...
subroutine hw3(r1,r2,s) ! in :hw:hw.f
real*8 :: r1
real*8 :: r2
real*8, intent(out) :: s
end subroutine hw3
end interface
end python module hw
|
 | Next step: run F2PY with the edited interface file:
f2py -c hw.pyf hw.f
|

Output arguments are always returned
 | Load the module and print its doc string:
>>> import hw
>>> print hw.__doc__
Functions:
hw1 = hw1(r1,r2)
hw2(r1,r2)
s = hw3(r1,r2)
Oops! hw3 takes only two arguments and returns s!
|
 | This is the ``Pythonic'' function style; input data are arguments, output data are returned
|
 | By default, F2PY treats all arguments as input
|
 | F2PY generates Pythonic interfaces, different from the original Fortran interfaces, so check out the module's doc string!
|

General adjustment of interfaces
 | Function with multiple input and output variables
subroutine somef(i1, i2, o1, o2, o3, o4, io1)
|
 |
input: i1, i2
|
 | output: o1, ..., o4
|
 | input and output: io1
|
 | Pythonic interface (as generated by F2PY):
o1, o2, o3, o4, io1 = somef(i1, i2, io1)
|

Specification of input/output arguments; .pyf file
 | In the interface file:
python module somemodule
interface
...
subroutine somef(i1, i2, o1, o2, o3, o4, io1)
real*8, intent(in) :: i1
real*8, intent(in) :: i2
real*8, intent(out) :: o1
real*8, intent(out) :: o2
real*8, intent(out) :: o3
real*8, intent(out) :: o4
real*8, intent(in,out) :: io1
end subroutine somef
...
end interface
end python module somemodule
|
 | Note: no intent implies intent(in)
|

Specification of input/output arguments; .f file
 | Instead of editing the interface file, we can add
special F2PY comments in the Fortran source code:
subroutine somef(i1, i2, o1, o2, o3, o4, io1)
real*8 i1, i2, o1, o2, o3, o4, io1
Cf2py intent(in) i1
Cf2py intent(in) i2
Cf2py intent(out) o1
Cf2py intent(out) o2
Cf2py intent(out) o3
Cf2py intent(out) o4
Cf2py intent(in,out) io1
|
 | Now a single F2PY command generates correct interface:
f2py -m hw -c hw.f
|

Specification of input/output arguments; .f90 file
 | With Fortran 90:
subroutine somef(i1, i2, o1, o2, o3, o4, io1)
real*8 i1, i2, o1, o2, o3, o4, io1
!f2py intent(in) i1
!f2py intent(in) i2
!f2py intent(out) o1
!f2py intent(out) o2
!f2py intent(out) o3
!f2py intent(out) o4
!f2py intent(in,out) io1
|
 | Now a single F2PY command generates correct interface:
f2py -m hw -c hw.f
|

Integration of Python and C
 | Let us implement the hw module in C:
#include <stdio.h>
#include <math.h>
#include <stdlib.h>
double hw1(double r1, double r2)
{
double s; s = sin(r1 + r2); return s;
}
void hw2(double r1, double r2)
{
double s; s = sin(r1 + r2);
printf("Hello, World! sin(%g+%g)=%g\n", r1, r2, s);
}
/* special version of hw1 where the result is an argument: */
void hw3(double r1, double r2, double *s)
{
*s = sin(r1 + r2);
}
|

Using F2PY
 | F2PY can also wrap C code if we specify the function signatures
as Fortran 90 modules
|
 | My procedure:
 | write the C functions as empty Fortran 77 functions or subroutines
|  | run F2PY on the Fortran specification to generate an interface file
|  | run F2PY with the interface file and the C source code
|
|

Step 1: Write Fortran 77 signatures
C file signatures.f
real*8 function hw1(r1, r2)
Cf2py intent(c) hw1
real*8 r1, r2
Cf2py intent(c) r1, r2
end
subroutine hw2(r1, r2)
Cf2py intent(c) hw2
real*8 r1, r2
Cf2py intent(c) r1, r2
end
subroutine hw3(r1, r2, s)
Cf2py intent(c) hw3
real*8 r1, r2, s
Cf2py intent(c) r1, r2
Cf2py intent(out) s
end

Step 2: Generate interface file
 | Run
Unix/DOS> f2py -m hw -h hw.pyf signatures.f
|
 | Result: hw.pyf
python module hw ! in
interface ! in :hw
function hw1(r1,r2) ! in :hw:signatures.f
intent(c) hw1
real*8 intent(c) :: r1
real*8 intent(c) :: r2
real*8 intent(c) :: hw1
end function hw1
...
subroutine hw3(r1,r2,s) ! in :hw:signatures.f
intent(c) hw3
real*8 intent(c) :: r1
real*8 intent(c) :: r2
real*8 intent(out) :: s
end subroutine hw3
end interface
end python module hw
|

Step 3: compile C code into extension module
 | Run
Unix/DOS> f2py -c hw.pyf hw.c
|
 | Test:
import hw
print hw.hw3(1.0,-1.0)
print hw.__doc__
|
 | One can either write the interface file by hand or write F77 code to generate, but for every C function the Fortran signature must be specified
|

Using SWIG
 | Wrappers to C and C++ codes can be automatically generated by SWIG
|
 | SWIG is more complicated to use than F2PY
|
 | First make a SWIG interface file
|
 | Then run SWIG to generate wrapper code
|
 | Then compile and link the C code and the wrapper code
|

SWIG interface file
 | The interface file contains C preprocessor directives and special SWIG directives:
/* file: hw.i */
%module hw
%{
/* include C header files necessary to compile the interface */
#include "hw.h"
%}
/* list functions to be interfaced: */
double hw1(double r1, double r2);
void hw2(double r1, double r2);
void hw3(double r1, double r2, double *s);
# or
%include "hw.h" /* make interface to all funcs in hw.h */
|

Making the module
 | Run SWIG (preferably in a subdirectory):
swig -python -I.. hw.i
|
 | SWIG generates wrapper code in
hw_wrap.c
|
 | Compile and link a shared library module:
gcc -I.. -O -I/some/path/include/python2.5 \
-c ../hw.c hw_wrap.c
gcc -shared -o _hw.so hw.o hw_wrap.o
Note the underscore prefix in _hw.so
|

A build script
 | Can automate the compile+link process
|
 | Can use Python to extract where Python.h resides (needed by any wrapper code)
swig -python -I.. hw.i
root=`python -c 'import sys; print sys.prefix'`
ver=`python -c 'import sys; print sys.version[:3]'`
gcc -O -I.. -I$root/include/python$ver -c ../hw.c hw_wrap.c
gcc -shared -o _hw.so hw.o hw_wrap.o
python -c "import hw" # test
(these statements are found in make_module_1.sh)
|
 | The module consists of two files: hw.py (which loads)
_hw.so
|

Building modules with Distutils (1)
 | Python has a tool, Distutils, for compiling and linking extension modules
|
 | First write a script setup.py:
import os
from distutils.core import setup, Extension
name = 'hw' # name of the module
version = 1.0 # the module's version number
swig_cmd = 'swig -python -I.. %s.i' % name
print 'running SWIG:', swig_cmd
os.system(swig_cmd)
sources = ['../hw.c', 'hw_wrap.c']
setup(name = name, version = version,
ext_modules = [Extension('_' + name, # SWIG requires _
sources,
include_dirs=[os.pardir])
])
|

Building modules with Distutils (2)
 | Now run
python setup.py build_ext
python setup.py install --install-platlib=.
python -c 'import hw' # test
|
 | Can install resulting module files in any directory
|
 | Use Distutils for professional distribution!
|

Testing the hw3 function
 | Recall hw3:
void hw3(double r1, double r2, double *s)
{
*s = sin(r1 + r2);
}
|
 | Test:
>>> from hw import hw3
>>> r1 = 1; r2 = -1; s = 10
>>> hw3(r1, r2, s)
>>> print s
10 # should be 0 (sin(1-1)=0)
Major problem - as in the Fortran case
|

Specifying input/output arguments
 | We need to adjust the SWIG interface file:
/* typemaps.i allows input and output pointer arguments to be
specified using the names INPUT, OUTPUT, or INOUT */
%include "typemaps.i"
void hw3(double r1, double r2, double *OUTPUT);
|
 | Now the usage from Python is
s = hw3(r1, r2)
|
 | Unfortunately, SWIG does not document this in doc strings
|

Other tools
 | SIP: tool for wrapping C++ libraries
|
 | Boost.Python: tool for wrapping C++ libraries
|
 | CXX: C++ interface to Python (Boost is a replacement)
|
 | Note: SWIG can generate interfaces to most scripting languages (Perl, Ruby, Tcl, Java, Guile, Mzscheme, ...)
|

Integrating Python with C++
 | SWIG supports C++
|
 | The only difference is when we run SWIG (-c++ option):
swig -python -c++ -I.. hw.i
# generates wrapper code in hw_wrap.cxx
|
 | Use a C++ compiler to compile and link:
root=`python -c 'import sys; print sys.prefix'`
ver=`python -c 'import sys; print sys.version[:3]'`
g++ -O -I.. -I$root/include/python$ver \
-c ../hw.cpp hw_wrap.cxx
g++ -shared -o _hw.so hw.o hw_wrap.o
|

Interfacing C++ functions (1)
 | This is like interfacing C functions, except that pointers are usual replaced by references
void hw3(double r1, double r2, double *s) // C style
{ *s = sin(r1 + r2); }
void hw4(double r1, double r2, double& s) // C++ style
{ s = sin(r1 + r2); }
|

Interfacing C++ functions (2)
 | Interface file (hw.i):
%module hw
%{
#include "hw.h"
%}
%include "typemaps.i"
%apply double *OUTPUT { double* s }
%apply double *OUTPUT { double& s }
%include "hw.h"
|
 | That's it!
|

Interfacing C++ classes
 | C++ classes add more to the SWIG-C story
|
 | Consider a class version of our Hello World module:
class HelloWorld
{
protected:
double r1, r2, s;
void compute(); // compute s=sin(r1+r2)
public:
HelloWorld();
~HelloWorld();
void set(double r1, double r2);
double get() const { return s; }
void message(std::ostream& out) const;
};
|
 | Goal: use this class as a Python class
|

Function bodies and usage
 | Function bodies:
void HelloWorld:: set(double r1_, double r2_)
{
r1 = r1_; r2 = r2_;
compute(); // compute s
}
void HelloWorld:: compute()
{ s = sin(r1 + r2); }
etc.
|
 | Usage:
HelloWorld hw;
hw.set(r1, r2);
hw.message(std::cout); // write "Hello, World!" message
|
 | Files: HelloWorld.h, HelloWorld.cpp
|

Adding a subclass
 | To illustrate how to handle class hierarchies, we add a subclass:
class HelloWorld2 : public HelloWorld
{
public:
void gets(double& s_) const;
};
void HelloWorld2:: gets(double& s_) const { s_ = s; }
i.e., we have a function with an output argument
|
 | Note: gets should return the value when called from Python
|
 | Files: HelloWorld2.h, HelloWorld2.cpp
|

SWIG interface file
/* file: hw.i */
%module hw
%{
/* include C++ header files necessary to compile the interface */
#include "HelloWorld.h"
#include "HelloWorld2.h"
%}
%include "HelloWorld.h"
%include "typemaps.i"
%apply double* OUTPUT { double& s }
%include "HelloWorld2.h"

Adding a class method
 | SWIG allows us to add class methods
|
 | Calling message with standard output (std::cout) is tricky from Python so we add a print method for printing to std.output
|
 | print coincides with Python's keyword print so we follow the convention of adding an underscore:
%extend HelloWorld {
void print_() { self->message(std::cout); }
}
|
 | This is basically C++ syntax, but self is used instead of this and \%extend HelloWorld is a SWIG directive
|
 | Make extension module:
swig -python -c++ -I.. hw.i
# compile HelloWorld.cpp HelloWorld2.cpp hw_wrap.cxx
# link HelloWorld.o HelloWorld2.o hw_wrap.o to _hw.so
|

Using the module
from hw import HelloWorld
hw = HelloWorld() # make class instance
r1 = float(sys.argv[1]); r2 = float(sys.argv[2])
hw.set(r1, r2) # call instance method
s = hw.get()
print "Hello, World! sin(%g + %g)=%g" % (r1, r2, s)
hw.print_()
hw2 = HelloWorld2() # make subclass instance
hw2.set(r1, r2)
s = hw.gets() # original output arg. is now return value
print "Hello, World2! sin(%g + %g)=%g" % (r1, r2, s)

Remark
 | It looks that the C++ class hierarchy is mirrored in Python
|
 | Actually, SWIG wraps a function interface to any class:
import _hw # use _hw.so directly
_hw.HelloWorld_set(r1, r2)
|
 | SWIG also makes a proxy class in hw.py, mirroring the
original C++ class:
import hw # use hw.py interface to _hw.so
c = hw.HelloWorld()
c.set(r1, r2) # calls _hw.HelloWorld_set(r1, r2)
|
 | The proxy class introduces overhead
|

Intro to GUI programming

Contents
 | Introductory GUI programming
|
 | Scientific Hello World examples
|
 | GUI for simviz1.py
|
 | GUI elements: text, input text, buttons, sliders, frames (for controlling
layout)
|

GUI toolkits callable from Python
Python has interfaces to the GUI toolkits
 | Tk (Tkinter)
|
 | Qt (PyQt)
|
 | wxWidgets (wxPython)
|
 | Gtk (PyGtk)
|
 | Java Foundation Classes (JFC) (java.swing in Jython)
|
 | Microsoft Foundation Classes (PythonWin)
|

Discussion of GUI toolkits
 | Tkinter has been the default Python GUI toolkit
|
 | Most Python installations support Tkinter
|
 | PyGtk, PyQt and wxPython are increasingly popular and more
sophisticated toolkits
|
 | These toolkits require huge C/C++ libraries (Gtk, Qt, wxWindows) to be installed on the user's machine
|
 | Some prefer to generate GUIs using an interactive designer tool,
which automatically generates calls to the GUI toolkit
|
 | Some prefer to program the GUI code (or automate that process)
|
 | It is very wise (and necessary) to learn some GUI programming even if you end up using a designer tool
|
 | We treat Tkinter (with extensions) here since it is so widely
available and simpler to use than its competitors
|
 | See doc.html for
links to literature on PyGtk, PyQt, wxPython and associated designer tools
|

More info
 | Ch. 6 in the course book
|
 | ``Introduction to Tkinter'' by Lundh (see doc.html)
|
 | Efficient working style: grab GUI code from examples
|
 | Demo programs:
$PYTHONSRC/Demo/tkinter
demos/All.py in the Pmw source tree
$scripting/src/gui/demoGUI.py
|

Tkinter, Pmw and Tix
 | Tkinter is an interface to the Tk package in C (for Tcl/Tk)
|
 | Megawidgets, built from basic Tkinter widgets, are available in Pmw (Python megawidgets) and Tix
|
 | Pmw is written in Python
|
 | Tix is written in C (and as Tk, aimed at Tcl users)
|
 | GUI programming becomes simpler and more modular by using classes; Python supports this programming style
|

Scientific Hello World GUI

 | Graphical user interface (GUI) for computing the sine of numbers
|
 | The complete window is made of widgets
(also referred to as windows)
|
 | Widgets from left to right:
 | a label with "Hello, World! The sine of"
|  | a text entry where the user can write a number
|  | pressing the button "equals" computes the sine of the number
|  | a label displays the sine value
|
|

The code (1)

#!/usr/bin/env python
from Tkinter import *
import math
root = Tk() # root (main) window
top = Frame(root) # create frame (good habit)
top.pack(side='top') # pack frame in main window
hwtext = Label(top, text='Hello, World! The sine of')
hwtext.pack(side='left')
r = StringVar() # special variable to be attached to widgets
r.set('1.2') # default value
r_entry = Entry(top, width=6, relief='sunken', textvariable=r)
r_entry.pack(side='left')

The code (2)
s = StringVar() # variable to be attached to widgets
def comp_s():
global s
s.set('%g' % math.sin(float(r.get()))) # construct string
compute = Button(top, text=' equals ', command=comp_s)
compute.pack(side='left')
s_label = Label(top, textvariable=s, width=18)
s_label.pack(side='left')
root.mainloop()

Structure of widget creation
 | A widget has a parent widget
|
 | A widget must be packed (placed in the parent widget) before it can appear visually
|
 | Typical structure:
widget = Tk_class(parent_widget,
arg1=value1, arg2=value2)
widget.pack(side='left')
|
 | Variables can be tied to the contents of, e.g., text entries, but only special Tkinter variables are legal:
StringVar, DoubleVar, IntVar
|

The event loop
 | No widgets are visible before we call the event loop:
root.mainloop()
|
 | This loop waits for user input (e.g. mouse clicks)
|
 | There is no predefined program flow after the event loop is invoked; the program just responds to events
|
 | The widgets define the event responses
|

Binding events

 | Instead of clicking "equals", pressing return in the entry window computes the sine value
# bind a Return in the .r entry to calling comp_s:
r_entry.bind('<Return>', comp_s)
|
 | One can bind any keyboard or mouse event to user-defined functions
|
 | We have also replaced the "equals" button by a straight label
|

Packing widgets
 | The pack command determines the placement of the widgets:
widget.pack(side='left')
This results in stacking widgets from left to right
|


Packing from top to bottom
 | Packing from top to bottom:
widget.pack(side='top')
results in
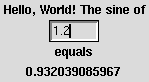
|
 | Values of side: left, right, top,
bottom
|

Lining up widgets with frames
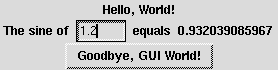
 | Frame: empty widget holding other widgets
(used to group widgets)
|
 | Make 3 frames, packed from top
|
 | Each frame holds a row of widgets
|
 | Middle frame: 4 widgets packed from left
|

Code for middle frame
# create frame to hold the middle row of widgets:
rframe = Frame(top)
# this frame (row) is packed from top to bottom:
rframe.pack(side='top')
# create label and entry in the frame and pack from left:
r_label = Label(rframe, text='The sine of')
r_label.pack(side='left')
r = StringVar() # variable to be attached to widgets
r.set('1.2') # default value
r_entry = Entry(rframe, width=6, relief='sunken', textvariable=r)
r_entry.pack(side='left')

Change fonts
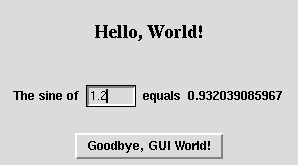
# platform-independent font name:
font = 'times 18 bold'
# or X11-style:
font = '-adobe-times-bold-r-normal-*-18-*-*-*-*-*-*-*'
hwtext = Label(hwframe, text='Hello, World!',
font=font)

Add space around widgets
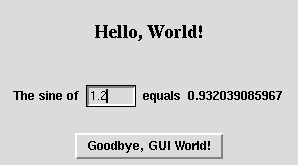
padx and pady adds space around widgets:
hwtext.pack(side='top', pady=20)
rframe.pack(side='top', padx=10, pady=20)

Changing colors and widget size
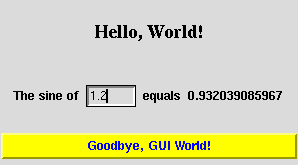
quit_button = Button(top,
text='Goodbye, GUI World!',
command=quit,
background='yellow',
foreground='blue')
quit_button.pack(side='top', pady=5, fill='x')
# fill='x' expands the widget throughout the available
# space in the horizontal direction

Translating widgets
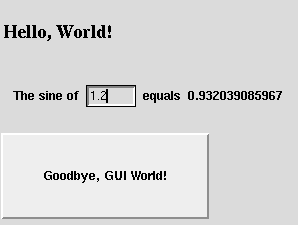
 | The anchor option can move widgets:
quit_button.pack(anchor='w')
# or 'center', 'nw', 's' and so on
# default: 'center'
|
 | ipadx/ipady: more space inside the widget
quit_button.pack(side='top', pady=5,
ipadx=30, ipady=30, anchor='w')
|

Learning about pack
Pack is best demonstrated through packdemo.tcl:
$scripting/src/tools/packdemo.tcl
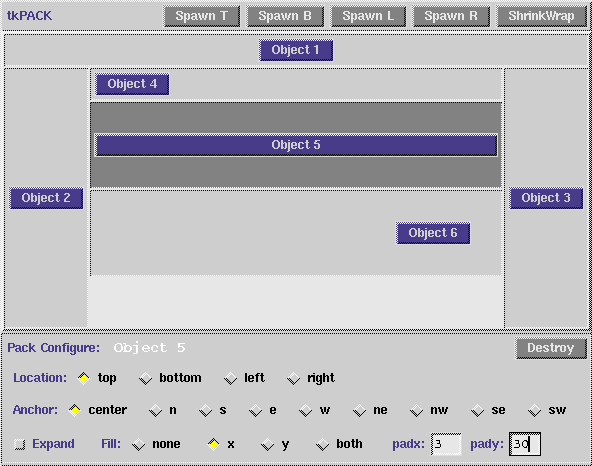

The grid geometry manager
 | Alternative to pack: grid
|
 | Widgets are organized in m times n cells, like a spreadsheet
|
 | Widget placement:
widget.grid(row=1, column=5)
|
 | A widget can span more than one cell
widget.grid(row=1, column=2, columnspan=4)
|

Basic grid options
 | Padding as with pack (padx, ipadx etc.)
|
 | sticky replaces anchor and fill
|

Example: Hello World GUI with grid
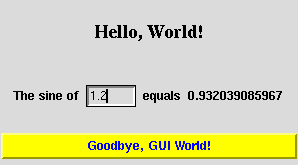
# use grid to place widgets in 3x4 cells:
hwtext.grid(row=0, column=0, columnspan=4, pady=20)
r_label.grid(row=1, column=0)
r_entry.grid(row=1, column=1)
compute.grid(row=1, column=2)
s_label.grid(row=1, column=3)
quit_button.grid(row=2, column=0, columnspan=4, pady=5,
sticky='ew')

The sticky option
 | sticky='w' means anchor='w'
(move to west)
|
 | sticky='ew' means fill='x'
(move to east and west)
|
 | sticky='news' means fill='both'
(expand in all dirs)
|

Configuring widgets (1)
 | So far: variables tied to text entry and result label
|
 | Another method:
 | ask text entry about its content
|  | update result label with configure
|
|
 | Can use configure to update any widget property
|

Configuring widgets (2)
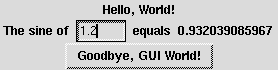
 | No variable is tied to the entry:
r_entry = Entry(rframe, width=6, relief='sunken')
r_entry.insert('end','1.2') # insert default value
r = float(r_entry.get())
s = math.sin(r)
s_label.configure(text=str(s))
|
 | Other properties can be configured:
s_label.configure(background='yellow')
|

Glade: a designer tool
 | With the basic knowledge of GUI programming, you may try out a
designer tool for interactive automatic generation of a GUI
|
 | Glade: designer tool for PyGtk
|
 | Gtk, PyGtk and Glade must be installed (not part of Python!)
|
 | See doc.html for introductions to Glade
|
 | Working style: pick a widget, place it in the GUI window,
open a properties dialog, set packing parameters, set callbacks
(signals in PyGtk), etc.
|
 | Glade stores the GUI in an XML file
|
 | The GUI is hence separate from the application code
|

GUI as a class
 | GUIs are conveniently implemented as classes
|
 | Classes in Python are similar to classes in Java and C++
|
 | Constructor: create and pack all widgets
|
 | Methods: called by buttons, events, etc.
|
 | Attributes: hold widgets, widget variables, etc.
|
 | The class instance can be used as an encapsulated GUI
component in other GUIs (like a megawidget)
|

The basics of Python classes
 | Declare a base class MyBase:
class MyBase:
def __init__(self,i,j): # constructor
self.i = i; self.j = j
def write(self): # member function
print 'MyBase: i=',self.i,'j=',self.j
|
 | self is a reference to this object
|
 | Data members are prefixed by self:
self.i, self.j
|
 | All functions take self as first argument in the
declaration, but not in the call
inst1 = MyBase(6,9); inst1.write()
|

Implementing a subclass
 | Class MySub is a subclass of MyBase:
class MySub(MyBase):
def __init__(self,i,j,k): # constructor
MyBase.__init__(self,i,j)
self.k = k;
def write(self):
print 'MySub: i=',self.i,'j=',self.j,'k=',self.k
|
 | Example:
# this function works with any object that has a write method:
def write(v): v.write()
# make a MySub instance
inst2 = MySub(7,8,9)
write(inst2) # will call MySub's write
|

Creating the GUI as a class (1)
class HelloWorld:
def __init__(self, parent):
# store parent
# create widgets as in hwGUI9.py
def quit(self, event=None):
# call parent's quit, for use with binding to 'q'
# and quit button
def comp_s(self, event=None):
# sine computation
root = Tk()
hello = HelloWorld(root)
root.mainloop()

Creating the GUI as a class (2)
class HelloWorld:
def __init__(self, parent):
self.parent = parent # store the parent
top = Frame(parent) # create frame for all class widgets
top.pack(side='top') # pack frame in parent's window
# create frame to hold the first widget row:
hwframe = Frame(top)
# this frame (row) is packed from top to bottom:
hwframe.pack(side='top')
# create label in the frame:
font = 'times 18 bold'
hwtext = Label(hwframe, text='Hello, World!', font=font)
hwtext.pack(side='top', pady=20)

Creating the GUI as a class (3)
# create frame to hold the middle row of widgets:
rframe = Frame(top)
# this frame (row) is packed from top to bottom:
rframe.pack(side='top', padx=10, pady=20)
# create label and entry in the frame and pack from left:
r_label = Label(rframe, text='The sine of')
r_label.pack(side='left')
self.r = StringVar() # variable to be attached to r_entry
self.r.set('1.2') # default value
r_entry = Entry(rframe, width=6, textvariable=self.r)
r_entry.pack(side='left')
r_entry.bind('<Return>', self.comp_s)
compute = Button(rframe, text=' equals ',
command=self.comp_s, relief='flat')
compute.pack(side='left')

Creating the GUI as a class (4)
self.s = StringVar() # variable to be attached to s_label
s_label = Label(rframe, textvariable=self.s, width=12)
s_label.pack(side='left')
# finally, make a quit button:
quit_button = Button(top, text='Goodbye, GUI World!',
command=self.quit,
background='yellow', foreground='blue')
quit_button.pack(side='top', pady=5, fill='x')
self.parent.bind('<q>', self.quit)
def quit(self, event=None):
self.parent.quit()
def comp_s(self, event=None):
self.s.set('%g' % math.sin(float(self.r.get())))

More on event bindings (1)
 | Event bindings call functions that take an event object as argument:
self.parent.bind('<q>', self.quit)
def quit(self,event): # the event arg is required!
self.parent.quit()
|
 | Button must call a quit function without arguments:
def quit():
self.parent.quit()
quit_button = Button(frame, text='Goodbye, GUI World!',
command=quit)
|

More on event bindings (1)
 | Here is aunified quit function that can be used with buttons and event bindings:
def quit(self, event=None):
self.parent.quit()
|
 | Keyword arguments and None as default value make Python programming effective!
|

A kind of calculator
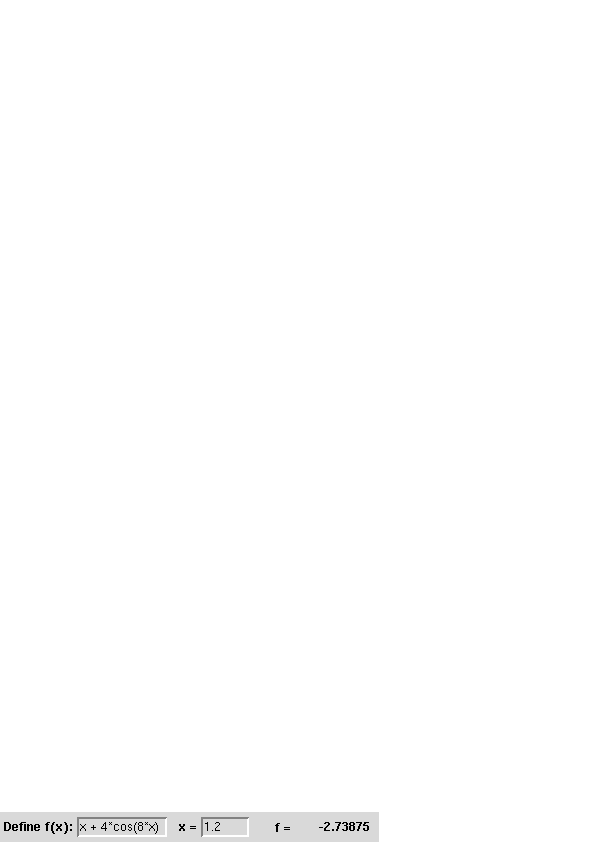
Label + entry + label + entry + button + label
# f_widget, x_widget are text entry widgets
f_txt = f_widget.get() # get function expression as string
x = float(x_widget.get()) # get x as float
#####
res = eval(f_txt) # turn f_txt expression into Python code
#####
label.configure(text='%g' % res) # display f(x)

Turn strings into code: eval and exec
 | eval(s) evaluates a Python expression s
eval('sin(1.2) + 3.1**8')
|
 | exec(s) executes the string s as Python code
s = 'x = 3; y = sin(1.2*x) + x**8'
exec(s)
|
 | Main application: get Python expressions from a GUI (no need to parse mathematical expressions if they follow the Python syntax!), build tailored code at run-time depending on input to the script
|

A GUI for simviz1.py
 | Recall simviz1.py: automating simulation and visualization of an oscillating system via a simple command-line interface
|
 | GUI interface:
|
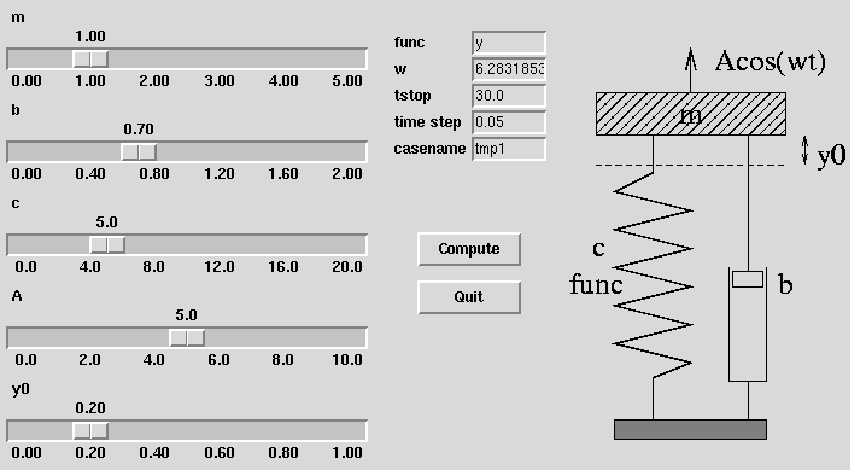

The code (1)
class SimVizGUI:
def __init__(self, parent):
"""build the GUI"""
self.parent = parent
...
self.p = {} # holds all Tkinter variables
self.p['m'] = DoubleVar(); self.p['m'].set(1.0)
self.slider(slider_frame, self.p['m'], 0, 5, 'm')
self.p['b'] = DoubleVar(); self.p['b'].set(0.7)
self.slider(slider_frame, self.p['b'], 0, 2, 'b')
self.p['c'] = DoubleVar(); self.p['c'].set(5.0)
self.slider(slider_frame, self.p['c'], 0, 20, 'c')

The code (2)
def slider(self, parent, variable, low, high, label):
"""make a slider [low,high] tied to variable"""
widget = Scale(parent, orient='horizontal',
from_=low, to=high, # range of slider
# tickmarks on the slider "axis":
tickinterval=(high-low)/5.0,
# the steps of the counter above the slider:
resolution=(high-low)/100.0,
label=label, # label printed above the slider
length=300, # length of slider in pixels
variable=variable) # slider value is tied to variable
widget.pack(side='top')
return widget
def textentry(self, parent, variable, label):
"""make a textentry field tied to variable"""
...

Layout
 | Use three frames: left, middle, right
|
 | Place sliders in the left frame
|
 | Place text entry fields in the middle frame
|
 | Place a sketch of the system in the right frame
|

The text entry field
 | Version 1 of creating a text field: straightforward packing of labels and entries in frames:
def textentry(self, parent, variable, label):
"""make a textentry field tied to variable"""
f = Frame(parent)
f.pack(side='top', padx=2, pady=2)
l = Label(f, text=label)
l.pack(side='left')
widget = Entry(f, textvariable=variable, width=8)
widget.pack(side='left', anchor='w')
return widget
|

The result is not good...
The text entry frames (f) get centered:
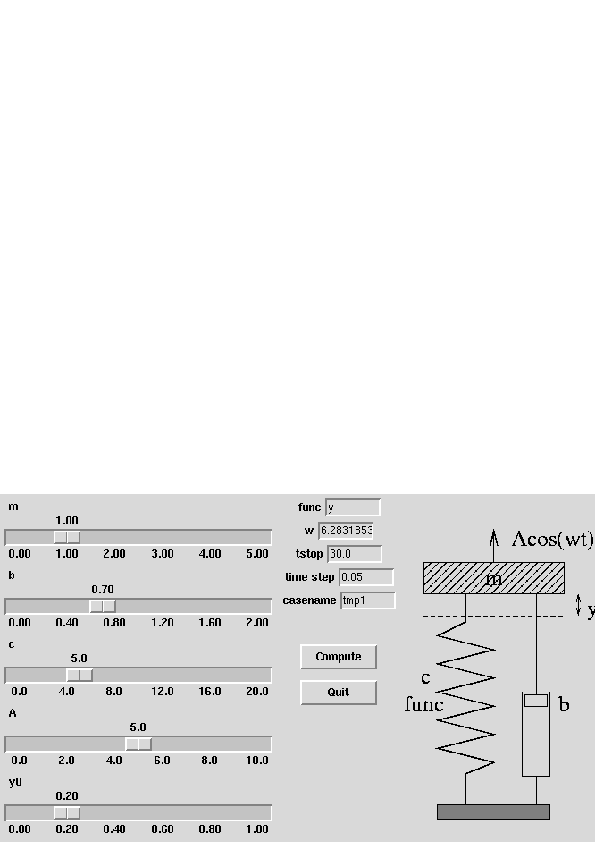
Ugly!

Improved text entry layout
 | Use the grid geometry manager to place labels and text entry fields in a spreadsheet-like fashion:
def textentry(self, parent, variable, label):
"""make a textentry field tied to variable"""
l = Label(parent, text=label)
l.grid(column=0, row=self.row_counter, sticky='w')
widget = Entry(parent, textvariable=variable, width=8)
widget.grid(column=1, row=self.row_counter)
self.row_counter += 1
return widget
|
 | You can mix the use of grid and pack, but not within the same
frame
|

The image
sketch_frame = Frame(self.parent)
sketch_frame.pack(side='left', padx=2, pady=2)
gifpic = os.path.join(os.environ['scripting'],
'src','gui','figs','simviz2.xfig.t.gif')
self.sketch = PhotoImage(file=gifpic)
# (images must be tied to a global or class variable!)
Label(sketch_frame,image=self.sketch).pack(side='top',pady=20)

Simulate and visualize buttons
 | Straight buttons calling a function
|
 | Simulate: copy code from simviz1.py
(create dir, create input file, run simulator)
|
 | Visualize: copy code from simviz1.py
(create file with Gnuplot commands, run Gnuplot)
|
Complete script: src/py/gui/simvizGUI2.py

Resizing widgets (1)
 | Example: display a file in a text widget
root = Tk()
top = Frame(root); top.pack(side='top')
text = Pmw.ScrolledText(top, ...
text.pack()
# insert file as a string in the text widget:
text.insert('end', open(filename,'r').read())
|
 | Problem: the text widget is not resized when the main window is resized
|
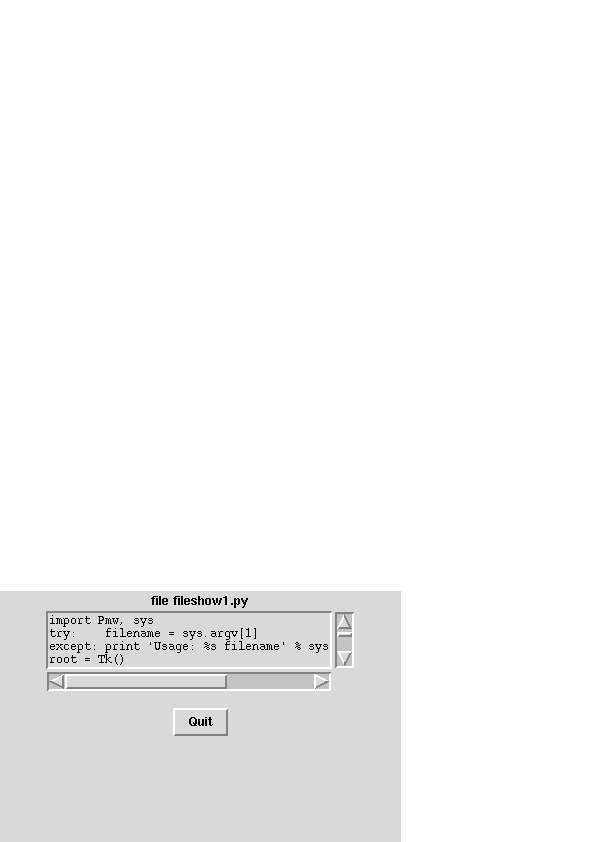

Resizing widgets (2)
 | Solution: combine the expand and fill options to pack:
text.pack(expand=1, fill='both')
# all parent widgets as well:
top.pack(side='top', expand=1, fill='both')
expand allows the widget to expand,
fill tells in which directions
the widget is allowed to expand
|
 | Try fileshow1.py and fileshow2.py!
|
 | Resizing is important for text, canvas and list widgets
|

Pmw demo program
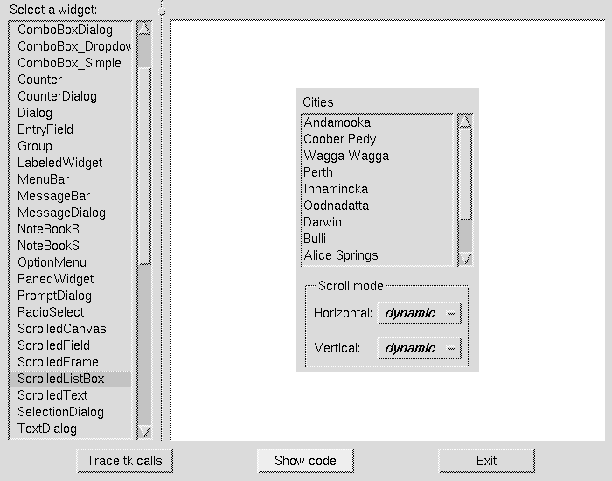
Very useful demo program in All.py (comes with Pmw)

Test/doc part of library files
 | A Python script can act both as a library file (module) and an executable test example
|
 | The test example is in a special end block
# demo program ("main" function) in case we run the script
# from the command line:
if __name__ == '__main__':
root = Tkinter.Tk()
Pmw.initialise(root)
root.title('preliminary test of ScrolledListBox')
# test:
widget = MyLibGUI(root)
root.mainloop()
|
 | Makes a built-in test for verification
|
 | Serves as documentation of usage
|

Numerical mixed-language programming

Contents
 | Migrating slow for loops over NumPy arrays to Fortran, C and C++
|
 | F2PY handling of arrays
|
 | Handwritten C and C++ modules
|
 | C++ class for wrapping NumPy arrays
|
 | C++ modules using SCXX
|
 | Pointer communication and SWIG
|
 | Efficiency considerations
|

More info
 | Ch. 5, 9 and 10 in the course book
|
 | F2PY manual
|
 | SWIG manual
|
 | Examples coming with the SWIG source code
|
 | Electronic Python documentation:
Extending and Embedding..., Python/C API
|
 | Python in a Nutshell
|
 | Python Essential Reference (Beazley)
|

Is Python slow for numerical computing?
 | Fill a NumPy array with function values:
n = 2000
a = zeros((n,n))
xcoor = arange(0,1,1/float(n))
ycoor = arange(0,1,1/float(n))
for i in range(n):
for j in range(n):
a[i,j] = f(xcoor[i], ycoor[j]) # f(x,y) = sin(x*y) + 8*x
|
 | Fortran/C/C++ version: (normalized) time 1.0
|
 | NumPy vectorized evaluation of f: time 3.0
|
 | Python loop version (version): time 140 (math.sin)
|
 | Python loop version (version): time 350 (numarray.sin)
|

Comments
 | Python loops over arrays are extremely slow
|
 | NumPy vectorization may be sufficient
|
 | However, NumPy vectorization may be inconvenient
- plain loops in Fortran/C/C++ are much easier
|
 | Write administering code in Python
|
 | Identify bottlenecks (via profiling)
|
 | Migrate slow Python code to Fortran, C, or C++
|
 | Python-Fortran w/NumPy arrays via F2PY: easy
|
 | Python-C/C++ w/NumPy arrays via SWIG: not that easy,
handwritten wrapper code is most common
|

Case: filling a grid with point values
 | Consider a rectangular 2D grid
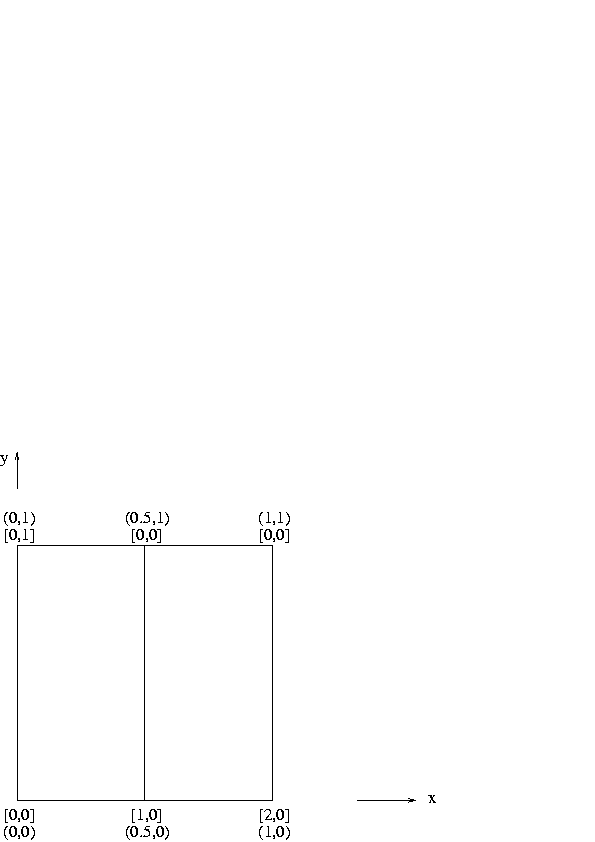
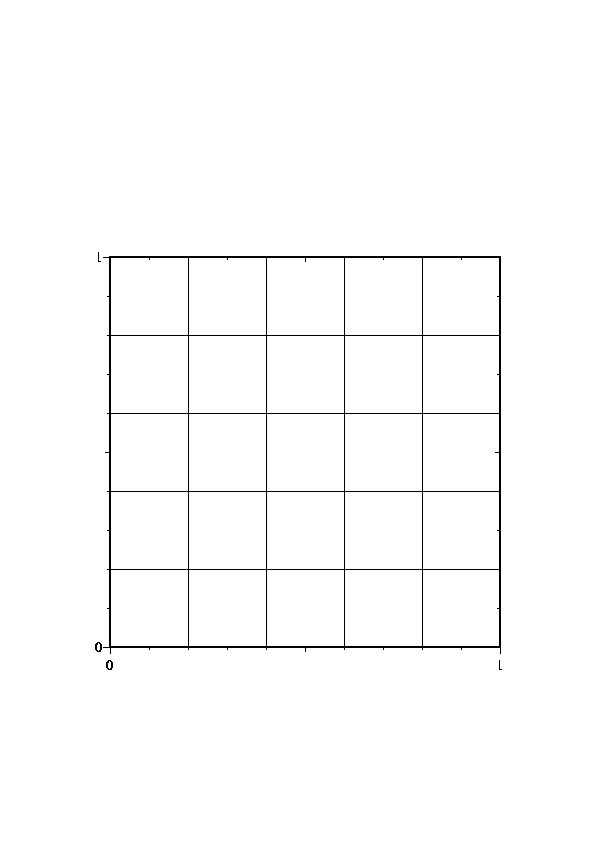
|
 | A NumPy array a[i,j] holds values at the grid points
|

Python object for grid data
 | Python class:
class Grid2D:
def __init__(self,
xmin=0, xmax=1, dx=0.5,
ymin=0, ymax=1, dy=0.5):
self.xcoor = sequence(xmin, xmax, dx)
self.ycoor = sequence(ymin, ymax, dy)
# make two-dim. versions of these arrays:
# (needed for vectorization in __call__)
self.xcoorv = self.xcoor[:,newaxis]
self.ycoorv = self.ycoor[newaxis,:]
def __call__(self, f):
# vectorized code:
return f(self.xcoorv, self.ycoorv)
|

Slow loop
 | Include a straight Python loop also:
class Grid2D:
....
def gridloop(self, f):
lx = size(self.xcoor); ly = size(self.ycoor)
a = zeros((lx,ly))
for i in range(lx):
x = self.xcoor[i]
for j in range(ly):
y = self.ycoor[j]
a[i,j] = f(x, y)
return a
|
 | Usage:
g = Grid2D(dx=0.01, dy=0.2)
def myfunc(x, y):
return sin(x*y) + y
a = g(myfunc)
i=4; j=10;
print 'value at (%g,%g) is %g' % (g.xcoor[i],g.ycoor[j],a[i,j])
|

Migrate gridloop to F77
class Grid2Deff(Grid2D):
def __init__(self,
xmin=0, xmax=1, dx=0.5,
ymin=0, ymax=1, dy=0.5):
Grid2D.__init__(self, xmin, xmax, dx, ymin, ymax, dy)
def ext_gridloop1(self, f):
"""compute a[i,j] = f(xi,yj) in an external routine."""
lx = size(self.xcoor); ly = size(self.ycoor)
a = zeros((lx,ly))
ext_gridloop.gridloop1(a, self.xcoor, self.ycoor, f)
return a
We can also migrate to C and C++ (done later)

F77 function
 | First try (typical attempt by a Fortran/C programmer):
subroutine gridloop1(a, xcoor, ycoor, nx, ny, func1)
integer nx, ny
real*8 a(0:nx-1,0:ny-1), xcoor(0:nx-1), ycoor(0:ny-1)
real*8 func1
external func1
integer i,j
real*8 x, y
do j = 0, ny-1
y = ycoor(j)
do i = 0, nx-1
x = xcoor(i)
a(i,j) = func1(x, y)
end do
end do
return
end
|
 | Note: float type in NumPy array must match real*8 or double precision in Fortran! (Otherwise F2PY will take a copy of the array a so the type matches that in the F77 code)
|

Making the extension module
 | Run F2PY:
f2py -m ext_gridloop -c gridloop.f
|
 | Try it from Python:
import ext_gridloop
ext_gridloop.gridloop1(a, self.xcoor, self.ycoor, myfunc,
size(self.xcoor), size(self.ycoor))
wrong results; a is not modified!
|
 | Reason: the gridloop1 function works on a copy a (because higher-dimensional arrays are stored differently in C/Python and Fortran)
|

Array storage in Fortran and C/C++
 | C and C++ has row-major storage
(two-dimensional arrays are stored row by row)
|
 | Fortran has column-major storage
(two-dimensional arrays are stored column by column)
|
 | Multi-dimensional arrays: first index has fastest variation in Fortran, last index has fastest variation in C and C++
|

Example: storing a 2x3 array
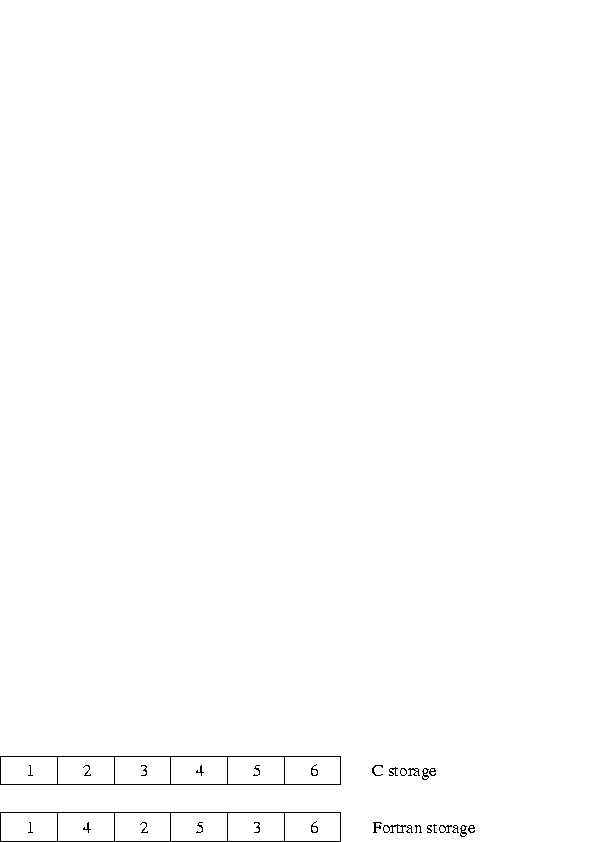
1 & 2 & 3
4 & 5 & 6
\]

F2PY and multi-dimensional arrays
 | F2PY-generated modules treat storage schemes transparently
|
 | If input array has C storage, a copy is taken, calculated with, and returned as output
|
 | F2PY needs to know whether arguments are input, output or both
|
 | To monitor (hidden) array copying, turn on the flag
f2py ... -DF2PY_REPORT_ON_ARRAY_COPY=1
|
 | In-place operations on NumPy arrays are possible in Fortran, but the default is to work on a copy, that is why our gridloop1 function does not work
|

Always specify input/output data
 | Insert Cf2py comments to tell that a is an output variable:
subroutine gridloop2(a, xcoor, ycoor, nx, ny, func1)
integer nx, ny
real*8 a(0:nx-1,ny-1), xcoor(0:nx-1), ycoor(0:ny-1), func1
external func1
Cf2py intent(out) a
Cf2py intent(in) xcoor
Cf2py intent(in) ycoor
Cf2py depend(nx,ny) a
|

gridloop2 seen from Python
 | F2PY generates this Python interface:
>>> import ext_gridloop
>>> print ext_gridloop.gridloop2.__doc__
gridloop2 - Function signature:
a = gridloop2(xcoor,ycoor,func1,[nx,ny,func1_extra_args])
Required arguments:
xcoor : input rank-1 array('d') with bounds (nx)
ycoor : input rank-1 array('d') with bounds (ny)
func1 : call-back function
Optional arguments:
nx := len(xcoor) input int
ny := len(ycoor) input int
func1_extra_args := () input tuple
Return objects:
a : rank-2 array('d') with bounds (nx,ny)
|
 | nx and ny are optional (!)
|

Handling of arrays with F2PY
 | Output arrays are returned and are not part of the argument list, as seen from Python
|
 | Need depend(nx,ny) a to specify that a is to be created with size nx, ny in the wrapper
|
 | Array dimensions are optional arguments (!)
class Grid2Deff(Grid2D):
...
def ext_gridloop2(self, f):
a = ext_gridloop.gridloop2(self.xcoor, self.ycoor, f)
return a
|
 | The modified interface is well documented in the doc strings generated by F2PY
|

Input/output arrays (1)
 | What if we really want to send a as argument and let F77 modify it?
def ext_gridloop1(self, f):
lx = size(self.xcoor); ly = size(self.ycoor)
a = zeros((lx,ly))
ext_gridloop.gridloop1(a, self.xcoor, self.ycoor, f)
return a
|
 | This is not Pythonic code, but it can be realized
|
 | 1. the array must have Fortran storage
|
 | 2. the array argument must be intent(inout)
(in general not recommended)
|

Input/output arrays (2)
 | F2PY generated modules has a function for checking if an array has column major storage (i.e., Fortran storage):
>>> a = zeros((n,n), order='Fortran')
>>> isfortran(a)
True
>>> a = asarray(a, order='C') # back to C storage
>>> isfortran(a)
False
|

Input/output arrays (3)
 | Fortran function:
subroutine gridloop1(a, xcoor, ycoor, nx, ny, func1)
integer nx, ny
real*8 a(0:nx-1,ny-1), xcoor(0:nx-1), ycoor(0:ny-1), func1
C call this function with an array a that has
C column major storage!
Cf2py intent(inout) a
Cf2py intent(in) xcoor
Cf2py intent(in) ycoor
Cf2py depend(nx, ny) a
|
 | Python call:
def ext_gridloop1(self, f):
lx = size(self.xcoor); ly = size(self.ycoor)
a = asarray(a, order='Fortran')
ext_gridloop.gridloop1(a, self.xcoor, self.ycoor, f)
return a
|

Storage compatibility requirements
 | Only when a has Fortran (column major) storage, the Fortran function works on a itself
|
 | If we provide a plain NumPy array, it has C (row major) storage, and the wrapper sends a copy to the Fortran function and transparently transposes the result
|
 | Hence, F2PY is very user-friendly, at a cost of some extra memory
|
 | The array returned from F2PY has Fortran (column major) storage
|

F2PY and storage issues
 | intent(out) a is the right specification; a should not be an argument in the Python call
|
 | F2PY wrappers will work on copies, if needed, and hide problems with different storage scheme in Fortran and C/Python
|
 | Python call:
a = ext_gridloop.gridloop2(self.xcoor, self.ycoor, f)
|

Caution
 | Find problems with this code (comp is a Fortran function in the extension module pde):
x = arange(0, 1, 0.01)
b = myfunc1(x) # compute b array of size (n,n)
u = myfunc2(x) # compute u array of size (n,n)
c = myfunc3(x) # compute c array of size (n,n)
dt = 0.05
for i in range(n)
u = pde.comp(u, b, c, i*dt)
|

About Python callbacks
 | It is convenient to specify the myfunc in Python
|
 | However, a callback to Python is costly, especially when done a large number of times (for every grid point)
|
 | Avoid such callbacks; vectorize callbacks
|
 | The Fortran routine should actually direct a back to Python (i.e., do nothing...) for a vectorized operation
|
 | Let's do this for illustration
|

Vectorized callback seen from Python
class Grid2Deff(Grid2D):
...
def ext_gridloop_vec(self, f):
"""Call extension, then do a vectorized callback to Python."""
lx = size(self.xcoor); ly = size(self.ycoor)
a = zeros((lx,ly))
a = ext_gridloop.gridloop_vec(a, self.xcoor, self.ycoor, f)
return a
def myfunc(x, y):
return sin(x*y) + 8*x
def myfuncf77(a, xcoor, ycoor, nx, ny):
"""Vectorized function to be called from extension module."""
x = xcoor[:,NewAxis]; y = ycoor[NewAxis,:]
a[:,:] = myfunc(x, y) # in-place modification of a
g = Grid2Deff(dx=0.2, dy=0.1)
a = g.ext_gridloop_vec(myfuncf77)

Vectorized callback from Fortran
subroutine gridloop_vec(a, xcoor, ycoor, nx, ny, func1)
integer nx, ny
real*8 a(0:nx-1,ny-1), xcoor(0:nx-1), ycoor(0:ny-1)
Cf2py intent(in,out) a
Cf2py intent(in) xcoor
Cf2py intent(in) ycoor
external func1
C fill array a with values taken from a Python function,
C do that without loop and point-wise callback, do a
C vectorized callback instead:
call func1(a, xcoor, ycoor, nx, ny)
C could work further with array a here...
return
end

Caution
 | What about this Python callback:
def myfuncf77(a, xcoor, ycoor, nx, ny):
"""Vectorized function to be called from extension module."""
x = xcoor[:,NewAxis]; y = ycoor[NewAxis,:]
a = myfunc(x, y)
|
 | a now refers to a new NumPy array; no in-place modification of the input argument
|

Avoiding callback by string-based if-else wrapper
 | Callbacks are expensive
|
 | Even vectorized callback functions degrades performace a bit
|
 | Alternative: implement ``callback'' in F77
|
 | Flexibility from the Python side: use a string to switch between the ``callback'' (F77) functions
a = ext_gridloop.gridloop2_str(self.xcoor, self.ycoor, 'myfunc')
F77 wrapper:
subroutine gridloop2_str(xcoor, ycoor, func_str)
character*(*) func_str
...
if (func_str .eq. 'myfunc') then
call gridloop2(a, xcoor, ycoor, nx, ny, myfunc)
else if (func_str .eq. 'f2') then
call gridloop2(a, xcoor, ycoor, nx, ny, f2)
...
|

Compiled callback function
 | Idea: if callback formula is a string, we could embed it in a Fortran function and call Fortran instead of Python
|
 | F2PY has a module for ``inline'' Fortran code specification and building
source = """
real*8 function fcb(x, y)
real*8 x, y
fcb = %s
return
end
""" % fstr
import f2py2e
f2py_args = "--fcompiler='Gnu' --build-dir tmp2 etc..."
f2py2e.compile(source, modulename='callback',
extra_args=f2py_args, verbose=True,
source_fn='sourcecodefile.f')
import callback
<work with the new extension module>
|

gridloop2 wrapper
 | To glue F77 gridloop2 and the F77 callback function, we make a gridloop2 wrapper:
subroutine gridloop2_fcb(a, xcoor, ycoor, nx, ny)
integer nx, ny
real*8 a(0:nx-1,ny-1), xcoor(0:nx-1), ycoor(0:ny-1)
Cf2py intent(out) a
Cf2py depend(nx,ny) a
real*8 fcb
external fcb
call gridloop2(a, xcoor, ycoor, nx, ny, fcb)
return
end
|
 | This wrapper and the callback function fc constitute the F77 source code, stored in source
|
 | The source calls gridloop2 so the module must be linked with the module containing gridloop2 (ext_gridloop.so)
|

Building the module on the fly
source = """
real*8 function fcb(x, y)
...
subroutine gridloop2_fcb(a, xcoor, ycoor, nx, ny)
...
""" % fstr
f2py_args = "--fcompiler='Gnu' --build-dir tmp2"\
" -DF2PY_REPORT_ON_ARRAY_COPY=1 "\
" ./ext_gridloop.so"
f2py2e.compile(source, modulename='callback',
extra_args=f2py_args, verbose=True,
source_fn='_cb.f')
import callback
a = callback.gridloop2_fcb(self.xcoor, self.ycoor)

gridloop2 could be generated on the fly
def ext_gridloop2_compile(self, fstr):
if not isinstance(fstr, str):
<error>
# generate Fortran source for gridloop2:
import f2py2e
source = """
subroutine gridloop2(a, xcoor, ycoor, nx, ny)
...
do j = 0, ny-1
y = ycoor(j)
do i = 0, nx-1
x = xcoor(i)
a(i,j) = %s
...
""" % fstr # no callback, the expression is hardcoded
f2py2e.compile(source, modulename='ext_gridloop2', ...)
def ext_gridloop2_v2(self):
import ext_gridloop2
return ext_gridloop2.gridloop2(self.xcoor, self.ycoor)

Extracting a pointer to the callback function
 | We can implement the callback function in Fortran, grab an F2PY-generated
pointer to this function and feed that as the func1 argument such that
Fortran calls Fortran and not Python
|
 | For a module m, the pointer to a function/subroutine f is reached as m.f._cpointer
def ext_gridloop2_fcb_ptr(self):
from callback import fcb
a = ext_gridloop.gridloop2(self.xcoor, self.ycoor,
fcb._cpointer)
return a
fcb is a Fortran implementation of the callback in an F2PY-generated
extension module callback
|

C implementation of the loop
 | Let us write the gridloop1 and gridloop2 functions in C
|
 | Typical C code:
void gridloop1(double** a, double* xcoor, double* ycoor,
int nx, int ny, Fxy func1)
{
int i, j;
for (i=0; i<nx; i++) {
for (j=0; j<ny; j++) {
a[i][j] = func1(xcoor[i], ycoor[j])
}
|
 | Problem: NumPy arrays use single pointers to data
|
 | The above function represents a as a double pointer (common in C for two-dimensional arrays)
|

Using F2PY to wrap the C function
 | Use single-pointer arrays
|
 | Write C function signature with Fortran 77 syntax
|
 | Use F2PY to generate an interface file
|
 | Use F2PY to compile the interface file and the C code
|

Step 0: The modified C function
ypedef double (*Fxy)(double x, double y);
#define index(a, i, j) a[j*ny + i]
void gridloop2(double *a, double *xcoor, double *ycoor,
int nx, int ny, Fxy func1)
{
int i, j;
for (i=0; i<nx; i++) {
for (j=0; j<ny; j++) {
index(a, i, j) = func1(xcoor[i], ycoor[j]);
}
}
}

Step 1: Fortran 77 signatures
C file: signatures.f
subroutine gridloop2(a, xcoor, ycoor, nx, ny, func1)
Cf2py intent(c) gridloop2
integer nx, ny
Cf2py intent(c) nx,ny
real*8 a(0:nx-1,0:ny-1), xcoor(0:nx-1), ycoor(0:ny-1), func1
external func1
Cf2py intent(c, out) a
Cf2py intent(in) xcoor, ycoor
Cf2py depend(nx,ny) a
C sample call of callback function:
real*8 x, y, r
real*8 func1
Cf2py intent(c) x, y, r, func1
x = 1
y = 1.51981721222
r = func1(x, y)
end

Step 3 and 4: Generate interface file and compile module
 | 3: Run
Unix/DOS> f2py -m ext_gridloop -h ext_gridloop.pyf signatures.f
|
 | 4: Run
Unix/DOS> f2py -c --fcompiler=Gnu --build-dir tmp1 \
-DF2PY_REPORT_ON_ARRAY_COPY=1 ext_gridloop.pyf gridloop.c
|
 | See
src/py/mixed/Grid2D/C/f2py
for all the involved files
|

Manual writing of extension modules
 | SWIG needs some non-trivial tweaking to handle NumPy arrays (i.e., the use of SWIG is much more complicated for array arguments than running F2PY)
|
 | We shall write a complete extension module by hand
|
 | We will need documentation of the Python C API (from Python's
electronic doc.) and the NumPy C API (from the NumPy book)
|
 | Source code files in
src/mixed/py/Grid2D/C/plain
|
 | Warning: manual writing of extension modules is very much more
complicated than using F2PY on Fortran or C code! You need to know C quite well...
|

NumPy objects as seen from C
NumPy objects are C structs with attributes:
 | int nd: no of indices (dimensions)
|
 | int dimensions[nd]: length of each dimension
|
 | char *data: pointer to data
|
 | int strides[nd]: no of bytes between two successive data elements for a fixed index
|
 | Access element (i,j) by
a->data + i*a->strides[0] + j*a->strides[1]
|

Creating new NumPy array in C
 | Allocate a new array:
PyObject * PyArray_FromDims(int n_dimensions,
int dimensions[n_dimensions],
int type_num);
PyArrayObject *a; int dims[2];
dims[0] = 10; dims[1] = 21;
a = (PyArrayObject *) PyArray_FromDims(2, dims, PyArray_DOUBLE);
|

Wrapping data in a NumPy array
 | Wrap an existing memory segment (with array data) in a NumPy array object:
PyObject * PyArray_FromDimsAndData(int n_dimensions,
int dimensions[n_dimensions],
int item_type,
char *data);
/* vec is a double* with 10*21 double entries */
PyArrayObject *a; int dims[2];
dims[0] = 10; dims[1] = 21;
a = (PyArrayObject *) PyArray_FromDimsAndData(2, dims,
PyArray_DOUBLE, (char *) vec);
Note: vec is a stream of numbers, now interpreted as a two-dimensional array, stored row by row
|

From Python sequence to NumPy array
 | Turn any relevant Python sequence type (list, type, array) into a NumPy array:
PyObject * PyArray_ContiguousFromObject(PyObject *object,
int item_type,
int min_dim,
int max_dim);
Use min_dim and max_dim as 0 to preserve the original dimensions of object
|
 | Application: ensure that an object is a NumPy array,
/* a_ is a PyObject pointer, representing a sequence
(NumPy array or list or tuple) */
PyArrayObject a;
a = (PyArrayObject *) PyArray_ContiguousFromObject(a_,
PyArray_DOUBLE, 0, 0);
a list, tuple or NumPy array a is now a NumPy array
|

Python interface
class Grid2Deff(Grid2D):
def __init__(self,
xmin=0, xmax=1, dx=0.5,
ymin=0, ymax=1, dy=0.5):
Grid2D.__init__(self, xmin, xmax, dx, ymin, ymax, dy)
def ext_gridloop1(self, f):
lx = size(self.xcoor); ly = size(self.ycoor)
a = zeros((lx,ly))
ext_gridloop.gridloop1(a, self.xcoor, self.ycoor, f)
return a
def ext_gridloop2(self, f):
a = ext_gridloop.gridloop2(self.xcoor, self.ycoor, f)
return a

gridloop1 in C; header
 | Transform PyObject argument tuple to NumPy arrays:
static PyObject *gridloop1(PyObject *self, PyObject *args)
{
PyArrayObject *a, *xcoor, *ycoor;
PyObject *func1, *arglist, *result;
int nx, ny, i, j;
double *a_ij, *x_i, *y_j;
/* arguments: a, xcoor, ycoor */
if (!PyArg_ParseTuple(args, "O!O!O!O:gridloop1",
&PyArray_Type, &a,
&PyArray_Type, &xcoor,
&PyArray_Type, &ycoor,
&func1)) {
return NULL; /* PyArg_ParseTuple has raised an exception */
}
|

gridloop1 in C; safety checks
if (a->nd != 2 || a->descr->type_num != PyArray_DOUBLE) {
PyErr_Format(PyExc_ValueError,
"a array is %d-dimensional or not of type float", a->nd);
return NULL;
}
nx = a->dimensions[0]; ny = a->dimensions[1];
if (xcoor->nd != 1 || xcoor->descr->type_num != PyArray_DOUBLE ||
xcoor->dimensions[0] != nx) {
PyErr_Format(PyExc_ValueError,
"xcoor array has wrong dimension (%d), type or length (%d)",
xcoor->nd,xcoor->dimensions[0]);
return NULL;
}
if (ycoor->nd != 1 || ycoor->descr->type_num != PyArray_DOUBLE ||
ycoor->dimensions[0] != ny) {
PyErr_Format(PyExc_ValueError,
"ycoor array has wrong dimension (%d), type or length (%d)",
ycoor->nd,ycoor->dimensions[0]);
return NULL;
}
if (!PyCallable_Check(func1)) {
PyErr_Format(PyExc_TypeError,
"func1 is not a callable function");
return NULL;
}

Callback to Python from C
 | Python functions can be called from C
|
 | Step 1: for each argument, convert C data to Python objects and collect these in a tuple
PyObject *arglist; double x, y;
/* double x,y -> tuple with two Python float objects: */
arglist = Py_BuildValue("(dd)", x, y);
|
 | Step 2: call the Python function
PyObject *result; /* return value from Python function */
PyObject *func1; /* Python function object */
result = PyEval_CallObject(func1, arglist);
|
 | Step 3: convert result to C data
double r; /* result is a Python float object */
r = PyFloat_AS_DOUBLE(result);
|

gridloop1 in C; the loop
for (i = 0; i < nx; i++) {
for (j = 0; j < ny; j++) {
a_ij = (double *)(a->data+i*a->strides[0]+j*a->strides[1]);
x_i = (double *)(xcoor->data + i*xcoor->strides[0]);
y_j = (double *)(ycoor->data + j*ycoor->strides[0]);
/* call Python function pointed to by func1: */
arglist = Py_BuildValue("(dd)", *x_i, *y_j);
result = PyEval_CallObject(func1, arglist);
*a_ij = PyFloat_AS_DOUBLE(result);
}
}
return Py_BuildValue(""); /* return None: */
}

Memory management
 | There is a major problem with our loop:
arglist = Py_BuildValue("(dd)", *x_i, *y_j);
result = PyEval_CallObject(func1, arglist);
*a_ij = PyFloat_AS_DOUBLE(result);
|
 | For each pass, arglist and result are dynamically allocated, but not destroyed
|
 | From the Python side, memory management is automatic
|
 | From the C side, we must do it ourself
|
 | Python applies reference counting
|
 | Each object has a number of references, one for each usage
|
 | The object is destroyed when there are no references
|

Reference counting
 | Increase the reference count:
Py_INCREF(myobj);
(i.e., I need this object, it cannot be deleted elsewhere)
|
 | Decrease the reference count:
Py_DECREF(myobj);
(i.e., I don't need this object, it can be deleted)
|

gridloop1; loop with memory management
for (i = 0; i < nx; i++) {
for (j = 0; j < ny; j++) {
a_ij = (double *)(a->data + i*a->strides[0] + j*a->strides[1]);
x_i = (double *)(xcoor->data + i*xcoor->strides[0]);
y_j = (double *)(ycoor->data + j*ycoor->strides[0]);
/* call Python function pointed to by func1: */
arglist = Py_BuildValue("(dd)", *x_i, *y_j);
result = PyEval_CallObject(func1, arglist);
Py_DECREF(arglist);
if (result == NULL) return NULL; /* exception in func1 */
*a_ij = PyFloat_AS_DOUBLE(result);
Py_DECREF(result);
}
}

gridloop1; more testing in the loop
 | We should check that allocations work fine:
arglist = Py_BuildValue("(dd)", *x_i, *y_j);
if (arglist == NULL) { /* out of memory */
PyErr_Format(PyExc_MemoryError,
"out of memory for 2-tuple);
|
 | The C code becomes quite comprehensive; much more testing than ``active'' statements
|

gridloop2 in C; header
gridloop2: as gridloop1, but array a is returned
static PyObject *gridloop2(PyObject *self, PyObject *args)
{
PyArrayObject *a, *xcoor, *ycoor;
int a_dims[2];
PyObject *func1, *arglist, *result;
int nx, ny, i, j;
double *a_ij, *x_i, *y_j;
/* arguments: xcoor, ycoor, func1 */
if (!PyArg_ParseTuple(args, "O!O!O:gridloop2",
&PyArray_Type, &xcoor,
&PyArray_Type, &ycoor,
&func1)) {
return NULL; /* PyArg_ParseTuple has raised an exception */
}
nx = xcoor->dimensions[0]; ny = ycoor->dimensions[0];

gridloop2 in C; macros
 | NumPy array code in C can be simplified using macros
|
 | First, a smart macro wrapping an argument in quotes:
#define QUOTE(s) # s /* turn s into string "s" */
|
 | Check the type of the array data:
#define TYPECHECK(a, tp) \
if (a->descr->type_num != tp) { \
PyErr_Format(PyExc_TypeError, \
"%s array is not of correct type (%d)", QUOTE(a), tp); \
return NULL; \
}
|
 | PyErr_Format is a flexible way of raising exceptions in C (must return NULL afterwards!)
|

gridloop2 in C; another macro
 | Check the length of a specified dimension:
#define DIMCHECK(a, dim, expected_length) \
if (a->dimensions[dim] != expected_length) { \
PyErr_Format(PyExc_ValueError, \
"%s array has wrong %d-dimension=%d (expected %d)", \
QUOTE(a),dim,a->dimensions[dim],expected_length); \
return NULL; \
}
|

gridloop2 in C; more macros
 | Check the dimensions of a NumPy array:
#define NDIMCHECK(a, expected_ndim) \
if (a->nd != expected_ndim) { \
PyErr_Format(PyExc_ValueError, \
"%s array is %d-dimensional, expected to be %d-dimensional",\
QUOTE(a), a->nd, expected_ndim); \
return NULL; \
}
|
 | Application:
NDIMCHECK(xcoor, 1); TYPECHECK(xcoor, PyArray_DOUBLE);
If xcoor is 2-dimensional, an exceptions is raised by NDIMCHECK:
exceptions.ValueError
xcoor array is 2-dimensional, but expected to be 1-dimensional
|

gridloop2 in C; indexing macros
 | Macros can greatly simplify indexing:
#define IND1(a, i) *((double *)(a->data + i*a->strides[0]))
#define IND2(a, i, j) \
*((double *)(a->data + i*a->strides[0] + j*a->strides[1]))
|
 | Application:
for (i = 0; i < nx; i++) {
for (j = 0; j < ny; j++) {
arglist = Py_BuildValue("(dd)", IND1(xcoor,i), IND1(ycoor,j));
result = PyEval_CallObject(func1, arglist);
Py_DECREF(arglist);
if (result == NULL) return NULL; /* exception in func1 */
IND2(a,i,j) = PyFloat_AS_DOUBLE(result);
Py_DECREF(result);
}
}
|

gridloop2 in C; the return array
 | Create return array:
a_dims[0] = nx; a_dims[1] = ny;
a = (PyArrayObject *) PyArray_FromDims(2, a_dims,
PyArray_DOUBLE);
if (a == NULL) {
printf("creating a failed, dims=(%d,%d)\n",
a_dims[0],a_dims[1]);
return NULL; /* PyArray_FromDims raises an exception */
}
|
 | After the loop, return a:
return PyArray_Return(a);
|

Registering module functions
 | The method table must always be present - it lists the functions that should be callable from Python:
static PyMethodDef ext_gridloop_methods[] = {
{"gridloop1", /* name of func when called from Python */
gridloop1, /* corresponding C function */
METH_VARARGS, /* ordinary (not keyword) arguments */
gridloop1_doc}, /* doc string for gridloop1 function */
{"gridloop2", /* name of func when called from Python */
gridloop2, /* corresponding C function */
METH_VARARGS, /* ordinary (not keyword) arguments */
gridloop2_doc}, /* doc string for gridloop1 function */
{NULL, NULL}
};
|
 | METH_KEYWORDS (instead of METH_VARARGS) implies that the function takes 3 arguments (self, args, kw)
|

Doc strings
static char gridloop1_doc[] = \
"gridloop1(a, xcoor, ycoor, pyfunc)";
static char gridloop2_doc[] = \
"a = gridloop2(xcoor, ycoor, pyfunc)";
static char module_doc[] = \
"module ext_gridloop:\n\
gridloop1(a, xcoor, ycoor, pyfunc)\n\
a = gridloop2(xcoor, ycoor, pyfunc)";

The required init function
PyMODINIT_FUNC initext_gridloop()
{
/* Assign the name of the module and the name of the
method table and (optionally) a module doc string:
*/
Py_InitModule3("ext_gridloop", ext_gridloop_methods, module_doc);
/* without module doc string:
Py_InitModule ("ext_gridloop", ext_gridloop_methods); */
import_array(); /* required NumPy initialization */
}

Building the module
root=`python -c 'import sys; print sys.prefix'`
ver=`python -c 'import sys; print sys.version[:3]'`
gcc -O3 -g -I$root/include/python$ver \
-I$scripting/src/C \
-c gridloop.c -o gridloop.o
gcc -shared -o ext_gridloop.so gridloop.o
# test the module:
python -c 'import ext_gridloop; print dir(ext_gridloop)'

A setup.py script
 | The script:
from distutils.core import setup, Extension
import os
name = 'ext_gridloop'
setup(name=name,
include_dirs=[os.path.join(os.environ['scripting'],
'src', 'C')],
ext_modules=[Extension(name, ['gridloop.c'])])
|
 | Usage:
python setup.py build_ext
python setup.py install --install-platlib=.
# test module:
python -c 'import ext_gridloop; print ext_gridloop.__doc__'
|

Using the module
 | The usage is the same as in Fortran, when viewed from Python
|
 | No problems with storage formats and unintended copying of a in gridloop1, or optional arguments; here we have full control of all details
|
 | gridloop2 is the ``right'' way to do it
|
 | It is much simpler to use Fortran and F2PY
|

Debugging
 | Things usually go wrong when you program...
|
 | Errors in C normally shows up as ``segmentation faults'' or ``bus error'' - no nice exception with traceback
|
 | Simple trick: run python under a debugger
unix> gdb `which python`
(gdb) run test.py
|
 | When the script crashes, issue the gdb command where for a traceback (if the extension module is compiled with -g you can see the line number of the line that triggered the error)
|
 | You can only see the traceback, no breakpoints, prints etc., but a tool, PyDebug, allows you to do this
|

Debugging example (1)
 | In src/py/mixed/Grid2D/C/plain/debugdemo there are some C files with errors
|
 | Try
./make_module_1.sh gridloop1
This scripts runs
../../../Grid2Deff.py verify1
which leads to a segmentation fault, implying that something is wrong in the C code (errors in the Python script shows up as exceptions with traceback)
|

1st debugging example (1)
 | Check that the extension module was compiled with debug mode on (usually the -g option to the C compiler)
|
 | Run python under a debugger:
unix> gdb `which python`
GNU gdb 6.0-debian
...
(gdb) run ../../../Grid2Deff.py verify1
Starting program: /usr/bin/python ../../../Grid2Deff.py verify1
...
Program received signal SIGSEGV, Segmentation fault.
0x40cdfab3 in gridloop1 (self=0x0, args=0x1) at gridloop1.c:20
20 if (!PyArg_ParseTuple(args, "O!O!O!O:gridloop1",
This is the line where something goes wrong...
|

1st debugging example (3)
(gdb) where
#0 0x40cdfab3 in gridloop1 (self=0x0, args=0x1) at gridloop1.c:20
#1 0x080fde1a in PyCFunction_Call ()
#2 0x080ab824 in PyEval_CallObjectWithKeywords ()
#3 0x080a9bde in Py_MakePendingCalls ()
#4 0x080aa76c in PyEval_EvalCodeEx ()
#5 0x080ab8d9 in PyEval_CallObjectWithKeywords ()
#6 0x080ab71c in PyEval_CallObjectWithKeywords ()
#7 0x080a9bde in Py_MakePendingCalls ()
#8 0x080ab95d in PyEval_CallObjectWithKeywords ()
#9 0x080ab71c in PyEval_CallObjectWithKeywords ()
#10 0x080a9bde in Py_MakePendingCalls ()
#11 0x080aa76c in PyEval_EvalCodeEx ()
#12 0x080acf69 in PyEval_EvalCode ()
#13 0x080d90db in PyRun_FileExFlags ()
#14 0x080d9d1f in PyRun_String ()
#15 0x08100c20 in _IO_stdin_used ()
#16 0x401ee79c in ?? ()
#17 0x41096bdc in ?? ()

1st debugging example (3)
 | What is wrong?
|
 | The import_array() call was removed, but the segmentation fault happended in the first call to a Python C function
|

2nd debugging example
 | Try
./make_module_1.sh gridloop2
and experience that
python -c 'import ext_gridloop; print dir(ext_gridloop); \
print ext_gridloop.__doc__'
ends with an exception
Traceback (most recent call last):
File "<string>", line 1, in ?
SystemError: dynamic module not initialized properly
|
 | This signifies that the module misses initialization
|
 | Reason: no Py_InitModule3 call
|

3rd debugging example (1)
 | Try
./make_module_1.sh gridloop3
|
 | Most of the program seems to work, but a segmentation fault occurs (according to gdb):
(gdb) where
(gdb) #0 0x40115d1e in mallopt () from /lib/libc.so.6
#1 0x40114d33 in malloc () from /lib/libc.so.6
#2 0x40449fb9 in PyArray_FromDimsAndDataAndDescr ()
from /usr/lib/python2.3/site-packages/Numeric/_numpy.so
...
#42 0x080d90db in PyRun_FileExFlags ()
#43 0x080d9d1f in PyRun_String ()
#44 0x08100c20 in _IO_stdin_used ()
#45 0x401ee79c in ?? ()
#46 0x41096bdc in ?? ()
|

3rd debugging example (2)
 | Next step: print out information
for (i = 0; i <= nx; i++) {
for (j = 0; j <= ny; j++) {
arglist = Py_BuildValue("(dd)", IND1(xcoor,i), IND1(ycoor,j));
result = PyEval_CallObject(func1, arglist);
IND2(a,i,j) = PyFloat_AS_DOUBLE(result);
#ifdef DEBUG
printf("a[%d,%d]=func1(%g,%g)=%g\n",i,j,
IND1(xcoor,i),IND1(ycoor,j),IND2(a,i,j));
#endif
}
}
|
 | Run
./make_module_1.sh gridloop3 -DDEBUG
|

3rd debugging example (3)
 | Loop debug output:
a[2,0]=func1(1,0)=1
f1...x-y= 3.0
a[2,1]=func1(1,1)=3
f1...x-y= 1.0
a[2,2]=func1(1,7.15113e-312)=1
f1...x-y= 7.66040480538e-312
a[3,0]=func1(7.6604e-312,0)=7.6604e-312
f1...x-y= 2.0
a[3,1]=func1(7.6604e-312,1)=2
f1...x-y= 2.19626564365e-311
a[3,2]=func1(7.6604e-312,7.15113e-312)=2.19627e-311
|
 | Ridiculous values (coordinates) and wrong indices reveal the problem: wrong upper loop limits
|

4th debugging example
 | Try
./make_module_1.sh gridloop4
and experience
python -c import ext_gridloop; print dir(ext_gridloop); \
print ext_gridloop.__doc__
Traceback (most recent call last):
File "<string>", line 1, in ?
ImportError: dynamic module does not define init function (initext_gridloop)
|
 | Eventuall we got a precise error message (the initext_gridloop was not implemented)
|

5th debugging example
 | Try
./make_module_1.sh gridloop5
and experience
python -c import ext_gridloop; print dir(ext_gridloop); \
print ext_gridloop.__doc__
Traceback (most recent call last):
File "<string>", line 1, in ?
ImportError: ./ext_gridloop.so: undefined symbol: mydebug
|
 | gridloop2 in gridloop5.c calls a function mydebug, but the function is not implemented (or linked)
|
 | Again, a precise ImportError helps detecting the problem
|

Summary of the debugging examples
 | Check that import_array() is called if the NumPy C API
is in use!
|
 | ImportError suggests wrong module initialization or missing required/user functions
|
 | You need experience to track down errors in the C code
|
 | An error in one place often shows up as an error in another place (especially indexing out of bounds or wrong memory handling)
|
 | Use a debugger (gdb) and print statements in the C code and the calling script
|
 | C++ modules are (almost) as error-prone as C modules
|

Next example
 | Implement the computational loop in a traditional C function
|
 | Aim: pretend that we have this loop already in a C library
|
 | Need to write a wrapper between this C function and Python
|
 | Could think of SWIG for generating the wrapper, but SWIG with NumPy arrays is a bit tricky - it is in fact simpler to write the wrapper by hand
|

Two-dim. C array as double pointer
 | C functions taking a two-dimensional array as argument will normally represent the array as a double pointer:
void gridloop1_C(double **a, double *xcoor, double *ycoor,
int nx, int ny, Fxy func1)
{
int i, j;
for (i=0; i<nx; i++) {
for (j=0; j<ny; j++) {
a[i][j] = func1(xcoor[i], ycoor[j]);
}
}
}
|
 | Fxy is a function pointer:
typedef double (*Fxy)(double x, double y);
|
 | An existing C library would typically work with multi-dim. arrays
and callback functions this way
|

Problems
 | How can we write wrapper code that sends NumPy array data to a C function as a double pointer?
|
 | How can we make callbacks to Python when the C function expects callbacks to standard C functions, represented as function pointers?
|
 | We need to cope with these problems to interface (numerical) C libraries!
|
src/mixed/py/Grid2D/C/clibcall

From NumPy array to double pointer
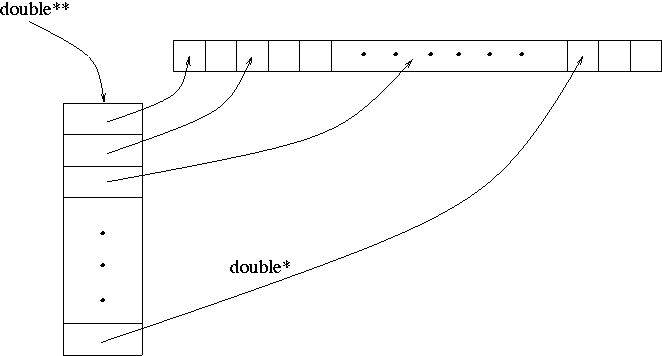
 | The wrapper code must allocate extra data:
double **app; double *ap;
ap = (double *) a->data; /* a is a PyArrayObject* pointer */
app = (double **) malloc(nx*sizeof(double*));
for (i = 0; i < nx; i++) {
app[i] = &(ap[i*ny]); /* point row no. i in a->data */
}
/* clean up when app is no longer needed: */ free(app);
|

Callback via a function pointer (1)
 | gridloop1_C calls a function like
double somefunc(double x, double y)
but our function is a Python object...
|
 | Trick: store the Python function in
PyObject* _pyfunc_ptr; /* global variable */
and make a ``wrapper'' for the call:
double _pycall(double x, double y)
{
/* perform call to Python function object in _pyfunc_ptr */
}
|

Callback via a function pointer (2)
 | Complete function wrapper:
double _pycall(double x, double y)
{
PyObject *arglist, *result;
arglist = Py_BuildValue("(dd)", x, y);
result = PyEval_CallObject(_pyfunc_ptr, arglist);
return PyFloat_AS_DOUBLE(result);
}
|
 | Initialize _pyfunc_ptr with the func1 argument supplied to the gridloop1 wrapper function
_pyfunc_ptr = func1; /* func1 is PyObject* pointer */
|

The alternative gridloop1 code (1)
static PyObject *gridloop1(PyObject *self, PyObject *args)
{
PyArrayObject *a, *xcoor, *ycoor;
PyObject *func1, *arglist, *result;
int nx, ny, i;
double **app;
double *ap, *xp, *yp;
/* arguments: a, xcoor, ycoor, func1 */
/* parsing without checking the pointer types: */
if (!PyArg_ParseTuple(args, "OOOO", &a, &xcoor, &ycoor, &func1))
{ return NULL; }
NDIMCHECK(a, 2); TYPECHECK(a, PyArray_DOUBLE);
nx = a->dimensions[0]; ny = a->dimensions[1];
NDIMCHECK(xcoor, 1); DIMCHECK(xcoor, 0, nx);
TYPECHECK(xcoor, PyArray_DOUBLE);
NDIMCHECK(ycoor, 1); DIMCHECK(ycoor, 0, ny);
TYPECHECK(ycoor, PyArray_DOUBLE);
CALLABLECHECK(func1);

The alternative gridloop1 code (2)
_pyfunc_ptr = func1; /* store func1 for use in _pycall */
/* allocate help array for creating a double pointer: */
app = (double **) malloc(nx*sizeof(double*));
ap = (double *) a->data;
for (i = 0; i < nx; i++) { app[i] = &(ap[i*ny]); }
xp = (double *) xcoor->data;
yp = (double *) ycoor->data;
gridloop1_C(app, xp, yp, nx, ny, _pycall);
free(app);
return Py_BuildValue(""); /* return None */
}

gridloop1 with C++ array object
 | Programming with NumPy arrays in C is much less convenient than programming with C++ array objects
SomeArrayClass a(10, 21);
a(1,2) = 3; // indexing
|
 | Idea: wrap NumPy arrays in a C++ class
|
 | Goal: use this class wrapper to simplify the gridloop1 wrapper
|
src/py/mixed/Grid2D/C++/plain

The C++ class wrapper (1)
class NumPyArray_Float
{
private:
PyArrayObject* a;
public:
NumPyArray_Float () { a=NULL; }
NumPyArray_Float (int n1, int n2) { create(n1, n2); }
NumPyArray_Float (double* data, int n1, int n2)
{ wrap(data, n1, n2); }
NumPyArray_Float (PyArrayObject* array) { a = array; }

The C++ class wrapper (2)
// redimension (reallocate) an array:
int create (int n1, int n2) {
int dim2[2]; dim2[0] = n1; dim2[1] = n2;
a = (PyArrayObject*) PyArray_FromDims(2, dim2, PyArray_DOUBLE);
if (a == NULL) { return 0; } else { return 1; } }
// wrap existing data in a NumPy array:
void wrap (double* data, int n1, int n2) {
int dim2[2]; dim2[0] = n1; dim2[1] = n2;
a = (PyArrayObject*) PyArray_FromDimsAndData(\
2, dim2, PyArray_DOUBLE, (char*) data);
}
// for consistency checks:
int checktype () const;
int checkdim (int expected_ndim) const;
int checksize (int expected_size1, int expected_size2=0,
int expected_size3=0) const;

The C++ class wrapper (3)
// indexing functions (inline!):
double operator() (int i, int j) const
{ return *((double*) (a->data +
i*a->strides[0] + j*a->strides[1])); }
double& operator() (int i, int j)
{ return *((double*) (a->data +
i*a->strides[0] + j*a->strides[1])); }
// extract dimensions:
int dim() const { return a->nd; } // no of dimensions
int size1() const { return a->dimensions[0]; }
int size2() const { return a->dimensions[1]; }
int size3() const { return a->dimensions[2]; }
PyArrayObject* getPtr () { return a; }
};

Using the wrapper class
static PyObject* gridloop2(PyObject* self, PyObject* args)
{
PyArrayObject *xcoor_, *ycoor_;
PyObject *func1, *arglist, *result;
/* arguments: xcoor, ycoor, func1 */
if (!PyArg_ParseTuple(args, "O!O!O:gridloop2",
&PyArray_Type, &xcoor_,
&PyArray_Type, &ycoor_,
&func1)) {
return NULL; /* PyArg_ParseTuple has raised an exception */
}
NumPyArray_Float xcoor (xcoor_); int nx = xcoor.size1();
if (!xcoor.checktype()) { return NULL; }
if (!xcoor.checkdim(1)) { return NULL; }
NumPyArray_Float ycoor (ycoor_); int ny = ycoor.size1();
// check ycoor dimensions, check that func1 is callable...
NumPyArray_Float a(nx, ny); // return array

The loop is straightforward
int i,j;
for (i = 0; i < nx; i++) {
for (j = 0; j < ny; j++) {
arglist = Py_BuildValue("(dd)", xcoor(i), ycoor(j));
result = PyEval_CallObject(func1, arglist);
a(i,j) = PyFloat_AS_DOUBLE(result);
}
}
return PyArray_Return(a.getPtr());

Reference counting
 | We have omitted a very important topic in Python-C programming: reference counting
|
 | Python has a garbage collection system based on reference counting
|
 | Each object counts the no of references to itself
|
 | When there are no more references, the object is automatically deallocated
|
 | Nice when used from Python, but in C we must program the reference counting manually
PyObject *obj;
...
Py_XINCREF(obj); /* new reference created */
...
Py_DECREF(obj); /* a reference is destroyed */
|

SCXX: basic ideas
 | Thin C++ layer on top of the Python C API
|
 | Each Python type (number, tuple, list, ...) is represented as a C++ class
|
 | The resulting code is quite close to Python
|
 | SCXX objects performs reference counting automatically
|

Example
#include <PWONumber.h> // class for numbers
#include <PWOSequence.h> // class for tuples
#include <PWOMSequence.h> // class for lists (immutable sequences)
void test_scxx()
{
double a_ = 3.4;
PWONumber a = a_; PWONumber b = 7;
PWONumber c; c = a + b;
PWOList list; list.append(a).append(c).append(b);
PWOTuple tp(list);
for (int i=0; i<tp.len(); i++) {
std::cout << "tp["<<i<<"]="<<double(PWONumber(tp[i]))<<" ";
}
std::cout << std::endl;
PyObject* py_a = (PyObject*) a; // convert to Python C struct
}

The similar code with Python C API
void test_PythonAPI()
{
double a_ = 3.4;
PyObject* a = PyFloat_FromDouble(a_);
PyObject* b = PyFloat_FromDouble(7);
PyObject* c = PyNumber_Add(a, b);
PyObject* list = PyList_New(0);
PyList_Append(list, a);
PyList_Append(list, c);
PyList_Append(list, b);
PyObject* tp = PyList_AsTuple(list);
int tp_len = PySequence_Length(tp);
for (int i=0; i<tp_len; i++) {
PyObject* qp = PySequence_GetItem(tp, i);
double q = PyFloat_AS_DOUBLE(qp);
std::cout << "tp[" << i << "]=" << q << " ";
}
std::cout << std::endl;
}
Note: reference counting is omitted

gridloop1 with SCXX
static PyObject* gridloop1(PyObject* self, PyObject* args_)
{
/* arguments: a, xcoor, ycoor */
try {
PWOSequence args (args_);
NumPyArray_Float a ((PyArrayObject*) ((PyObject*) args[0]));
NumPyArray_Float xcoor ((PyArrayObject*) ((PyObject*) args[1]));
NumPyArray_Float ycoor ((PyArrayObject*) ((PyObject*) args[2]));
PWOCallable func1 (args[3]);
// work with a, xcoor, ycoor, and func1
...
return PWONone();
}
catch (PWException e) { return e; }
}

Error checking
 | NumPyArray_Float objects are checked using their member functions (checkdim, etc.)
|
 | SCXX objects also have some checks:
if (!func1.isCallable()) {
PyErr_Format(PyExc_TypeError,
"func1 is not a callable function");
return NULL;
}
|

The loop over grid points
int i,j;
for (i = 0; i < nx; i++) {
for (j = 0; j < ny; j++) {
PWOTuple arglist(Py_BuildValue("(dd)", xcoor(i), ycoor(j)));
PWONumber result(func1.call(arglist));
a(i,j) = double(result);
}
}

The Weave tool (1)
 | Weave is an easy-to-use tool for inlining C++ snippets in Python codes
|
 | A quick demo shows its potential
class Grid2Deff:
...
def ext_gridloop1_weave(self, fstr):
"""Migrate loop to C++ with aid of Weave."""
from scipy import weave
# the callback function is now coded in C++
# (fstr must be valid C++ code):
extra_code = r"""
double cppcb(double x, double y) {
return %s;
}
""" % fstr
|

The Weave tool (2)
 | The loops: inline C++ with Blitz++ array syntax:
code = r"""
int i,j;
for (i=0; i<nx; i++) {
for (j=0; j<ny; j++) {
a(i,j) = cppcb(xcoor(i), ycoor(j));
}
}
"""
|

The Weave tool (3)
 | Compile and link the extra code extra_code
and the main code (loop) code:
nx = size(self.xcoor); ny = size(self.ycoor)
a = zeros((nx,ny))
xcoor = self.xcoor; ycoor = self.ycoor
err = weave.inline(code, ['a', 'nx', 'ny', 'xcoor', 'ycoor'],
type_converters=weave.converters.blitz,
support_code=extra_code, compiler='gcc')
return a
|
 | Note that we pass the names of the Python objects we want to
access in the C++ code
|
 | Weave is smart enough to avoid recompiling the code if it has not changed since last compilation
|

Exchanging pointers in Python code
 | When interfacing many libraries, data must be grabbed from one code and fed into another
|
 | Example: NumPy array to/from some C++ data class
|
 | Idea: make filters, converting one data to another
|
 | Data objects are represented by pointers
|
 | SWIG can send pointers back and forth without needing to wrap the whole underlying data object
|
 | Let's illustrate with an example!
|

MyArray: some favorite C++ array class
 | Say our favorite C++ array class is MyArray
template< typename T >
class MyArray
{
public:
T* A; // the data
int ndim; // no of dimensions (axis)
int size[MAXDIM]; // size/length of each dimension
int length; // total no of array entries
...
};
|
 | We can work with this class from Python without needing to SWIG the class (!)
|
 | We make a filter class converting a NumPy array (pointer) to/from a MyArray object (pointer)
|
src/py/mixed/Grid2D/C++/convertptr

Filter between NumPy array and C++ class
class Convert_MyArray
{
public:
Convert_MyArray();
// borrow data:
PyObject* my2py (MyArray<double>& a);
MyArray<double>* py2my (PyObject* a);
// copy data:
PyObject* my2py_copy (MyArray<double>& a);
MyArray<double>* py2my_copy (PyObject* a);
// print array:
void dump(MyArray<double>& a);
// convert Py function to C/C++ function calling Py:
Fxy set_pyfunc (PyObject* f);
protected:
static PyObject* _pyfunc_ptr; // used in _pycall
static double _pycall (double x, double y);
};

Typical conversion function
PyObject* Convert_MyArray:: my2py(MyArray<double>& a)
{
PyArrayObject* array = (PyArrayObject*) \
PyArray_FromDimsAndData(a.ndim, a.size, PyArray_DOUBLE,
(char*) a.A);
if (array == NULL) {
return NULL; /* PyArray_FromDimsAndData raised exception */
}
return PyArray_Return(array);
}

Version with data copying
PyObject* Convert_MyArray:: my2py_copy(MyArray<double>& a)
{
PyArrayObject* array = (PyArrayObject*) \
PyArray_FromDims(a.ndim, a.size, PyArray_DOUBLE);
if (array == NULL) {
return NULL; /* PyArray_FromDims raised exception */
}
double* ad = (double*) array->data;
for (int i = 0; i < a.length; i++) {
ad[i] = a.A[i];
}
return PyArray_Return(array);
}

Ideas
 | SWIG Convert_MyArray
|
 | Do not SWIG MyArray
|
 | Write numerical C++ code using MyArray
(or use a library that already makes use of MyArray)
|
 | Convert pointers (data) explicitly in the Python code
|

gridloop1 in C++
void gridloop1(MyArray<double>& a,
const MyArray<double>& xcoor,
const MyArray<double>& ycoor,
Fxy func1)
{
int nx = a.shape(1), ny = a.shape(2);
int i, j;
for (i = 0; i < nx; i++) {
for (j = 0; j < ny; j++) {
a(i,j) = func1(xcoor(i), ycoor(j));
}
}
}

Calling C++ from Python (1)
 | Instead of just calling
ext_gridloop.gridloop1(a, self.xcoor, self.ycoor, func)
return a
as before, we need some explicit conversions:
# a is a NumPy array
# self.c is the conversion module (class Convert_MyArray)
a_p = self.c.py2my(a)
x_p = self.c.py2my(self.xcoor)
y_p = self.c.py2my(self.ycoor)
f_p = self.c.set_pyfunc(func)
ext_gridloop.gridloop1(a_p, x_p, y_p, f_p)
return a # a_p and a share data!
|

Calling C++ from Python (2)
 | In case we work with copied data, we must copy both ways:
a_p = self.c.py2my_copy(a)
x_p = self.c.py2my_copy(self.xcoor)
y_p = self.c.py2my_copy(self.ycoor)
f_p = self.c.set_pyfunc(func)
ext_gridloop.gridloop1(a_p, x_p, y_p, f_p)
a = self.c.my2py_copy(a_p)
return a
|
 |
Note: final a is not the same a object as we started with
|

SWIG'ing the filter class
 | C++ code: convert.h/.cpp + gridloop.h/.cpp
|
 | SWIG interface file:
/* file: ext_gridloop.i */
%module ext_gridloop
%{
/* include C++ header files needed to compile the interface */
#include "convert.h"
#include "gridloop.h"
%}
%include "convert.h"
%include "gridloop.h"
|
 | Important: call NumPy's import_array (here in Convert_MyArray constructor)
|
 | Run SWIG:
swig -python -c++ -I. ext_gridloop.i
|
 | Compile and link shared library module
|

setup.py
import os
from distutils.core import setup, Extension
name = 'ext_gridloop'
swig_cmd = 'swig -python -c++ -I. %s.i' % name
os.system(swig_cmd)
sources = ['gridloop.cpp','convert.cpp','ext_gridloop_wrap.cxx']
setup(name=name,
ext_modules=[Extension('_' + name, # SWIG requires _
sources=sources,
include_dirs=[os.curdir])])

Manual alternative
swig -python -c++ -I. ext_gridloop.i
root=`python -c 'import sys; print sys.prefix'`
ver=`python -c 'import sys; print sys.version[:3]'`
g++ -I. -O3 -g -I$root/include/python$ver \
-c convert.cpp gridloop.cpp ext_gridloop_wrap.cxx
g++ -shared -o _ext_gridloop.so \
convert.o gridloop.o ext_gridloop_wrap.o

Summary
We have implemented several versions of gridloop1 and gridloop2:
 | Fortran subroutines, working on Fortran arrays, automatically wrapped by F2PY
|
 | Hand-written C extension module, working directly on NumPy array structs in C
|
 | Hand-written C wrapper to a C function, working on standard C arrays (incl. double pointer)
|
 | Hand-written C++ wrapper, working on a C++ class wrapper for NumPy arrays
|
 | As last point, but simplified wrapper utilizing SCXX
|
 | C++ functions based on MyArray, plus C++ filter for pointer conversion, wrapped by SWIG
|

Comparison
 | What is the most convenient approach in this case?
Fortran!
|
 | If we cannot use Fortran, which solution is attractive?
C++, with classes allowing higher-level programming
|
 | To interface a large existing library, the filter idea and exchanging pointers is attractive (no need to SWIG the whole library)
|
 | When using the Python C API extensively, SCXX simplifies life
|

Efficiency
 | Which alternative is computationally most efficient?
Fortran, but C/C++ is quite close -- no significant difference between all the C/C++ versions
|
 | Too bad: the (point-wise) callback to Python destroys the efficiency of the extension module!
|
 | Pure Python script w/NumPy is much more efficient...
|
 | Nevertheless: this is a pedagogical case teaching you how to migrate/interface numerical code
|

Efficiency test: 1100x1100 grid
language function func1 argument CPU time
F77 gridloop1 F77 function with formula 1.0
C++ gridloop1 C++ function with formula 1.07
Python Grid2D.__call__ vectorized numpy myfunc 1.5
Python Grid2D.gridloop myfunc w/math.sin 120
Python Grid2D.gridloop myfunc w/numpy.sin 220
F77 gridloop1 myfunc w/math.sin 40
F77 gridloop1 myfunc w/numpy.sin 180
F77 gridloop2 myfunc w/math.sin 40
F77 gridloop_vec2 vectorized myfunc 2.7
F77 gridloop2_str F77 myfunc 1.1
F77 gridloop_noalloc (no alloc. as in pure C++) 1.0
C gridloop1 myfunc w/math.sin 38
C gridloop2 myfunc w/math.sin 38
C++ (with class NumPyArray) had the same numbers as C

Conclusions about efficiency
 | math.sin is much faster than numpy.sin for scalar expressions
|
 | Callbacks to Python are extremely expensive
|
 | Python+NumPy is 1.5 times slower than pure Fortran
|
 | C and C++ run equally fast
|
 | C++ w/MyArray was only 7\% slower than pure F77
|
Minimize the no of callbacks to Python!

More F2PY features
 | Hide work arrays (i.e., allocate in wrapper):
subroutine myroutine(a, b, m, n, w1, w2)
integer m, n
real*8 a(m), b(n), w1(3*n), w2(m)
Cf2py intent(in,hide) w1
Cf2py intent(in,hide) w2
Cf2py intent(in,out) a
Python interface:
a = myroutine(a, b)
|
 | Reuse work arrays in subsequent calls (cache):
subroutine myroutine(a, b, m, n, w1, w2)
integer m, n
real*8 a(m), b(n), w1(3*n), w2(m)
Cf2py intent(in,hide,cache) w1
Cf2py intent(in,hide,cache) w2
|

Other tools
 | Pyfort for Python-Fortran integration
(does not handle F90/F95, not as simple as F2PY)
|
 | SIP: tool for wrapping C++ libraries
|
 | Boost.Python: tool for wrapping C++ libraries
|
 | CXX: C++ interface to Python (Boost is a replacement)
|
 | Note: SWIG can generate interfaces to most scripting languages (Perl, Ruby, Tcl, Java, Guile, Mzscheme, ...)
|

Quick Python review

Python info
 | doc.html is the resource portal for the course; load it into a web browser from
http://www.ifi.uio.no/~inf3330/scripting/doc.html
and make a bookmark
|
 | doc.html has links to the electronic Python documentation, F2PY, SWIG, Numeric/numarray, and lots of things used in the course
|
 | The course book ``Python scripting for computational science'' (the PDF version is fine for searching)
|
 | Python in a Nutshell (by Martelli)
|
 | Programming Python 2nd ed. (by Lutz)
|
 | Python Essential Reference (Beazley)
|
 | Quick Python Book
|

Electronic Python documentation
 | Python Tutorial
|
 | Python Library Reference (start with the index!)
|
 | Python Reference Manual (less used)
|
 | Extending and Embedding the Python Interpreter
|
 | Quick references from doc.html
|
 | pydoc anymodule, pydoc anymodule.anyfunc
|

Python variables
 | Variables are not declared
|
 | Variables hold references to objects of any type
a = 3 # reference to an int object containing 3
a = 3.0 # reference to a float object containing 3.0
a = '3.' # reference to a string object containing '3.'
a = ['1', 2] # reference to a list object containing
# a string '1' and an integer 2
|
 | Test for a variable's type:
if isinstance(a, int): # int?
if isinstance(a, (list, tuple)): # list or tuple?
|

Common types
 | Numbers: int, float, complex
|
 | Sequences: str (string), list, tuple, NumPy array
|
 | Mappings: dict (dictionary/hash)
|
 | User-defined type in terms of a class
|

Numbers
 | Integer, floating-point number, complex number
a = 3 # int
a = 3.0 # float
a = 3 + 0.1j # complex (3, 0.1)
|

List and tuple
 | List:
a = [1, 3, 5, [9.0, 0]] # list of 3 ints and a list
a[2] = 'some string'
a[3][0] = 0 # a is now [1,3,5,[0,0]]
b = a[0] # b refers first element in a
|
 | Tuple (``constant list''):
a = (1, 3, 5, [9.0, 0]) # tuple of 3 ints and a list
a[3] = 5 # illegal! (tuples are const/final)
|
 | Traversing list/tuple:
for item in a: # traverse list/tuple a
# item becomes, 1, 3, 5, and [9.0,0]
|

Dictionary
 | Making a dictionary:
a = {'key1': 'some value', 'key2': 4.1}
a['key1'] = 'another string value'
a['key2'] = [0, 1] # change value from float to string
a['another key'] = 1.1E+7 # add a new (key,value) pair
|
 |
Important: no natural sequence of (key,value) pairs!
|
 | Traversing dictionaries:
for key in some_dict:
# process key and corresponding value in some_dict[key]
|

Strings
 | Strings apply different types of quotes
s = 'single quotes'
s = "double quotes"
s = """triple quotes are
used for multi-line
strings
"""
s = r'raw strings start with r and backslash \ is preserved'
s = '\t\n' # tab + newline
s = r'\t\n' # a string with four characters: \t\n
|
 | Some useful operations:
if sys.platform.startswith('win'): # Windows machine?
...
file = infile[:-3] + '.gif' # string slice of infile
answer = answer.lower() # lower case
answer = answer.replace(' ', '_')
words = line.split()
|

NumPy arrays
 | Efficient arrays for numerical computing
from Numeric import * # classical, widely used module
from numarray import * # alternative version
|
 |
a = array([[1, 4], [2, 1]], Float) # 2x2 array from list
a = zeros((n,n), Float) # nxn array with 0
|
 | Indexing and slicing:
for i in xrange(a.shape[0]):
for j in xrange(a.shape[1]):
a[i,j] = ...
b = a[0,:] # reference to 1st row
b = a[:,1] # reference to 2nd column
|
 | Avoid loops and indexing, use operations that compute with whole arrays at once (in efficient C code)
|

Mutable and immutable types
 | Mutable types allow in-place modifications
>>> a = [1, 9, 3.2, 0]
>>> a[2] = 0
>>> a
[1, 9, 0, 0]
Types: list, dictionary, NumPy arrays, class instances
|
 | Immutable types do not allow in-place modifications
>>> s = 'some string containing x'
>>> s[-1] = 'y' # try to change last character - illegal!
TypeError: object doesn't support item assignment
>>> a = 5
>>> b = a # b is a reference to a (integer 5)
>>> a = 9 # a becomes a new reference
>>> b # b still refers to the integer 5
5
Types: numbers, strings
|

Operating system interface
 | Run arbitrary operating system command:
cmd = 'myprog -f -g 1.0 < input'
failure, output = commands.getstatusoutput(cmd)
|
 | Use commands.getstatsoutput for running applications
|
 | Use Python (cross platform) functions for listing files, creating directories, traversing file trees, etc.
psfiles = glob.glob('*.ps') + glob.glob('*.eps')
allfiles = os.listdir(os.curdir)
os.mkdir('tmp1'); os.chdir('tmp1')
print os.getcwd() # current working dir.
def size(arg, dir, files):
for file in files:
fullpath = os.path.join(dir,file)
s = os.path.getsize(fullpath)
arg.append((fullpath, s)) # save name and size
name_and_size = []
os.path.walk(os.curdir, size, name_and_size)
|

Files
 | Open and read:
f = open(filename, 'r')
filestr = f.read() # reads the whole file into a string
lines = f.readlines() # reads the whole file into a list of lines
for line in f: # read line by line
<process line>
while True: # old style, more flexible reading
line = f.readline()
if not line: break
<process line>
f.close()
|
 | Open and write:
f = open(filename, 'w')
f.write(somestring)
f.writelines(list_of_lines)
print >> f, somestring
|

Functions
 | Two types of arguments: positional and keyword
def myfync(pos1, pos2, pos3, kw1=v1, kw2=v2):
...
3 positional arguments, 2 keyword arguments (keyword=default-value)
|
 | Input data are arguments, output variables are returned as a tuple
def somefunc(i1, i2, i3, io1):
"""i1,i2,i3: input, io1: input and output"""
...
o1 = ...; o2 = ...; o3 = ...; io1 = ...
...
return o1, o2, o3, io1
|

Example: a grep script (1)
 | Find a string in a series of files:
grep.py 'Python' *.txt *.tmp
|
 | Python code:
def grep_file(string, filename):
res = {} # result: dict with key=line no. and value=line
f = open(filename, 'r')
line_no = 1
for line in f:
#if line.find(string) != -1:
if re.search(string, line):
res[line_no] = line
line_no += 1
|

Example: a grep script (2)
 | Let us put the previous function in a file grep.py
|
 | This file defines a module grep that we can import
|
 | Main program:
import sys, re, glob, grep
grep_res = {}
string = sys.argv[1]
for filespec in sys.argv[2:]:
for filename in glob.glob(filespec):
grep_res[filename] = grep.grep(string, filename)
# report:
for filename in grep_res:
for line_no in grep_res[filename]:
print '%-20s.%5d: %s' % (filename, line_no,
grep_res[filename][line_no])
|
%

Modules

Interactive Python
| Just write python in a terminal window to get an
interactive Python shell:
>>> 1269*1.24
1573.5599999999999
>>> import os; os.getcwd()
'/home/hpl/work/scripting/trunk/lectures'
>>> len(os.listdir('modules'))
60
|
| We recommend to use IPython as interactive shell
Unix/DOS> ipython
In [1]: 1+1
Out[1]: 2
|

IPython and the Python debugger
| Scripts can be run from IPython:
In [1]:run scriptfile arg1 arg2 ...
e.g.,
In [1]:run datatrans2.py .datatrans_infile tmp1
|
| IPython is integrated with Python's pdb debugger
|
| pdb can be automatically invoked when an exception occurs:
In [29]:%pdb on # invoke pdb automatically
In [30]:run datatrans2.py infile tmp2
|

More on debugging
| This happens when the infile name is wrong:
/home/work/scripting/src/py/intro/datatrans2.py
7 print "Usage:",sys.argv[0], "infile outfile"; sys.exit(1)
8
----> 9 ifile = open(infilename, 'r') # open file for reading
10 lines = ifile.readlines() # read file into list of lines
11 ifile.close()
IOError: [Errno 2] No such file or directory: 'infile'
> /home/work/scripting/src/py/intro/datatrans2.py(9)?()
-> ifile = open(infilename, 'r') # open file for reading
(Pdb) print infilename
infile
|